Identification and Measurement of Multidimensional Relative Poverty of Chinese Rural Adults Considering Climate Factors
- 1School of Business Administration, Zhongnan University of Economics and Law, Wuhan, China
- 2College of Management, Sichuan Agricultural University, Chengdu, China
The climate conditions in different regions of China are different, resulting in uneven climate resources owned by residents. It is important to design a comprehensive evaluation method to measure the multidimensional relative poverty (MRP) status and differences in rural areas considering climate factors from the micro-level. With adults as the research object, imitating multidimensional poverty index (MPI) and other indexes, and referring to the relative poverty lines in Britain, Australia, and other countries, this study considers the housing sunshine level and air quality of the living environment, which can reflect the superposition of economy and climate, in the dimension of human settlements environment, and establishes an indicator system of MRP in rural China. Using the Chinese General Social Survey data in 2018 and the A-F method to measure the indicator poverty rate, multidimensional relative poverty index (MRPI), and indicator contribution rate, this study evaluates the MRP in rural China including climate factors. The results show that the poverty rate of sunshine level and air quality indicator in North China is the highest in China, and the MRPI is the lowest. In North China, the sunshine level and air quality indicator poverty rate are 17.47% and 53.01%, respectively. MRPI under K = 1 standard is 0.1182. It shows that the indicator system can identify the typical phenomenon that highly industrialized economic development may negatively affect the environment. MRP alleviation should focus on coordinated governance of the economy, education, health, and the human settlements, we should establish a climate emergency plan for joint prevention and control with the meteorological department, set afforestation protection areas, set climate-related building standards such as sunshine times of rural houses, and improve and upgrade the energy use in rural areas to achieve the harmonious development of the society, economy, and environment and the high-quality life pursued by rural residents.
1 Introduction
The Chinese mainland is vast, located in the northern hemisphere, with a wide latitude of coastline. Moreover, the terrain is different in height and topography; the combination of sunshine, air temperature, precipitation, and air circulation is diverse. For China’s rural areas, sunshine conditions, as a climatic factor, affect all aspects of farmers’ production and life, such as physical health, food crop cultivation, and household income from agricultural production and operation. The sunshine resources of rural residents are differentiated in the whole country. Compared with rural residents living in the South or the plains, those in northern China or mountainous areas can enjoy relatively fewer sunshine resources. Moreover, there are differences in the spatial distribution of traditional leading industries in different regions of the Chinese mainland, and the degree of pollution such as fog and haze in the local environment also varies (Luo and Li, 2018). The air pollution represented by haze has varying degrees of impact on the local living environment, including air quality and sunshine level, and exacerbated the inequality of climate resources owned by residents in different regions. Therefore, poverty research needs to consider climate factors to reveal the multidimensional poverty differences including climate resources.
After the elimination of absolute poverty in China in 2020, the focus of poverty research will shift from relative poverty in a single economic dimension to multidimensional relative poverty (Pan and Yan, 2020; Wang and Feng, 2020; Zhong and Lin, 2020; Wang and Sun, 2021), the abbreviation of multidimensional relative poverty for the latter is MRP. Existing MRP studies focus on the economy, education, health, or living standards, and pay little attention to climatic factors such as sunshine, as well as cannot identify some MRP populations affected by climate. Therefore, it is necessary to change the previous method of measuring poverty with a single income indicator and consider climate factors in the identification, measurement, and governance of MRP.
The poverty status of rural family members is not “homogeneous” (Wu et al., 2013), There are significant differences in the degree, depth, and duration of poverty among the elderly, adults, and minors (Xiong and Song, 2018). The most accurate poverty identification, measurement, and governance should be detailed from the family to the individual level. First of all, according to China’s census data, China’s rural population at the end of 2018, 2019, and 2020 was 541.08, 525.82 and 509.79 million respectively, And the population aged 15–64 accounted for 68.6% of the total population in 2020. The proportion of the population aged 20–59 in 2018 and 2019 was 60.17% and 59.97%, respectively. The number of rural adults aged 19–59 is not only large but also a high proportion. Rural adults are the core group of primary concern in poverty research. Secondly, most of the existing micro-recognition objects of rural poverty are families (Liu and Wang, 2020; Pan and Yan, 2020; Wang and Feng, 2020), and a few are refined to adult male or female individuals (Chen, 2020; Wang and Liu, 2020; Zhang, 2020), or special groups of adults, such as migrant workers (Yang and Zhuang, 2021). Taking family as the object of identification can only indirectly represent the poverty status of adults. The poverty status of the adult population cannot be fully presented if males or females or specific adults are identified. And lastly, adults’ income is the main source of the family economy, and their development reflects the MRP status of the entire family to a certain extent. Therefore, taking adult individuals as the poverty identification object can identify and measure the inequality within rural families, and refine the existing MRP measurement scheme as the main poverty identification object. Referring to the age definition of adults and elderly groups in Chinese laws, adults in this paper are defined as those whose age is greater than or equal to 18 years old and less than or equal to 59 years old.
Imitating the multi-dimensional poverty index (MPI) and other indexes, and referring to the relative poverty measurement schemes of China, the UK, and Australia, this paper attempts to use the latest 2018 survey data of the Chinese General Social Survey (the abbreviation for the latter is CGSS) to build an indicator system for rural adult MRP measurement based on the individual level. Moreover, it aims to compare and analyze the status and characteristics of rural adult MRP, find out the key indicators that lead to the MRP of rural adults, and provide a theoretical basis for improving the policy.
2 Literature Review and Theoretical Basis
2.1 Literature Review
Most of the existing academic research about climate poverty focuses on the climate’s impact on poverty (Leichenko and Silva, 2014; Hallegatte and Rozenberg, 2017; Barbier and Hochard, 2018). Haines and Ebi (2019) found that climate change has an adverse impact on human health and health systems. Climate change will also affect household income. Arouri et al. (2015) used fixed-effect regression to estimate the impact of natural disasters on the welfare and poverty of rural households in Vietnam and found three types of disasters, including storms, floods, and droughts, had negative impacts on household income and expenditure. In addition, some scholars have studied the impact of climate change on migration (Cattaneo and Peri, 2016; Marotzke et al., 2020), food production (Ahmed et al., 2011; Tigchelaar et al., 2018), and labor productivity (Burke et al., 2015).
Sen’s (1999) feasible capability theory states that poverty needs to be measured from the aspects of individual feasible capability as well as freedom, including health, education, public service, spirit, and other dimensions, which instill a theoretical foundation for integrating climate factors into multidimensional poverty research. After this, the international research on multidimensional poverty paid more attention to individual development and subjective feelings and emphasized the importance of the living environment. Currently, the multidimensional poverty of families or individuals at the micro-level is mostly studied by combining non-monetary indicators such as education, health, and living environment with monetary indicators such as the economy (Alkire and Apablaza, 2016; Chen et al., 2017; He et al., 2017; Pérez-Cirera et al., 2017; Li S. et al., 2020; OPHI, 2020). The multidimensional poverty indicator system in some studies considered climate factors. Kahlan et al. (2021) set up a climate-related indicator system including “disaster preparedness” and “people affected by drought” to measure the multi-dimensional poverty of Iranian families. Zhang et al. (2019) considered China’s photovoltaic poverty alleviation areas as the object and included sunshine time and other indicators in the identification scope of multidimensional poverty. Yin et al. (2017) took into account the climate-related index of natural disasters in the multidimensional poverty indicator system of remote poverty alleviation and relocated families in China. Zhou et al. (2021) considered 124,000 poverty-stricken villages identified by China’s targeted poverty alleviation at the end of 2013 as the research object, and selected climate-related indicators such as temperature change, precipitation change, and natural disasters to measure multi-dimensional poverty in villages.
After China eliminated absolute poverty in 2020, multidimensional poverty was transformed into MRP. Xu et al. (2021) selected climate-related indicators such as precipitation and used counties of 31 provinces (municipalities) in China as the research object to measure the MRP at the macro level. Wei and Zhang (2021) set a disaster area indicator reflecting climate factors to measure MRP in Rural Areas of China.
Generally, the existing research began to include some climate factors in the identification and measurement of multidimensional poverty but it was mainly aimed at special scenarios such as disasters and photovoltaics, and there is a lack of poverty research in normalized scenarios. Few articles directly consider normal climate indicators such as sunshine and air quality when constructing a multidimensional relative poverty index, the abbreviation of multidimensional relative poverty index for the latter is MRPI. The research objects include counties or villages at the macro level and families at the micro level, which are not detailed at the individual level. Therefore, based on the existing academic achievements, this paper considers rural adults as the research object, integrates climate factors into the MRP indicator system, and considers the normalized living environment after the superposition of the economy and climate as the scenario to measure and reveal the MRP status and regional differences of Chinese rural adults under the joint action of economy and climate.
2.2 Theoretical Basis
As the literature review shows, the theoretical analysis of the formation mechanism of MRP in Rural China is fragmented at present. However, the mechanism of MRP among rural adults in China can be sorted out from existing literature. The existing literature on MRP involves four dimensions: economic, education, human settlements, and health. Among them, capital scarcity and low investment efficiency are important causes of economic poverty in China’s rural areas (Huang et al., 2016). An income gap in the process of economic growth also leads to economic poverty in China’s rural families (He, 2018). The formation of educational poverty is caused by the low level of human capital (Cheng et al., 2016), the uncertainty of the return on education investment (Gustafsson and Li, 2004), and the unequal distribution of education resources (He, 2018). Sunshine level, air pollution (Duan and Wang, 2020), geographical location of housing, and other factors together lead to poverty in China’s rural human settlements (Zhang and Jin, 2006). The reason why climate factors should be considered in the dimension of human settlements is that a livable environment cannot be separated from clean air, sufficient sunshine, appropriate temperature and humidity, and other factors closely related to climate. The temperature and humidity of the living environment largely depend on the sunshine level. China’s “Urban Residential District Planning and Design Standard” clearly stipulates that the sunshine time of houses shall not be less than 1 hour. In addition, a high-quality living environment also needs clean air. In terms of health poverty, the unequal distribution of medical resources in rural China and the lack of health knowledge among rural residents lead to health poverty (He, 2018; Wang and Liu, 2019).
The MRP of rural adults in China is dynamic, which is mainly manifested in three aspects: time, region, and population. In terms of time, the MRP of rural adults in China changes over time. In terms of regions, China can be divided into seven regions: Northeast, North, Northwest, East, Central, South, and Southwest. The MRP of these seven regions not only varies but also changes. In terms of population, the poverty degree of the MRP population will change. If the MRP degree of rural adults increases, the non-poverty population may change into an MRP population. If the MRP of rural adults in China is alleviated, the MRP population will be transformed into a non-poverty population.
3 MRP Identification Standard of Chinese Rural Adults Considering Climate Factors
Sen (1999) believes that poverty not only needs to be considered from economic dimensions such as income but also needs to find out whether people’s capability is deprived. Therefore, the cognition of poverty needs to be increased from a single “income” dimension to a multidimensional dimension such as education opportunities and health level, which also requires that poverty measurement methods adapt to the needs of multi-dimensional measurement. The World Development Report released by the World Bank in 2000 defines poverty as the deprivation of welfare. Poverty not only refers to material deprivation but also includes low levels of education and health. People gradually realize that poverty is a complex and comprehensive social phenomenon, in addition to income, poverty also involves the lack of multiple dimensions such as education, health, housing, and public goods (Ding, 2014). China’s poverty alleviation standard for the poor is “Two Assurances and Three Guarantees, that is, assured food and clothing, and guaranteed education, medical treatment, and housing. By 2020, China eliminated the population in absolute poverty, and the basic needs such as food, clothing, education, medical treatment, and housing of rural residents have been guaranteed. The expectations of rural residents for a better life are diversified, multi-level, and multifaceted: rural residents are looking forward to better education, more satisfactory income, higher level of medical and health services, more comfortable living conditions, more beautiful environment, and higher quality living environment. Therefore, along with the dimensions of economy, health, and education, this paper also adds human settlements including climate factors to more comprehensively evaluate the MRP status of rural adults in China.
3.1 Economic Dimension
Based on the simple and easy to operate income proportion method first proposed by Townsend (1979), the EU, OECD, and other regional, international organizations and countries generally set the identification standard of relative poverty as a proportion of average income or median income in practice (Li et al., 2021). Taking high-income countries such as the UK and Australia as examples, the relative poverty standard adopted by the UK is 60% of the median income (JRF, 2020), and that by Australia is 50% and 60% of the median household disposable income (Davidson et al., 2018). Most Chinese scholars believe that the relative poverty line of income should be set to be 40%, 50%, or 60% of the median per capita income (Shen and Li, 2020; Zhang and Duan, 2020). The proportion of median income adopted as the identification standard of relative poverty depends on a country’s income level and the government’s willingness to alleviate relative poverty; for China, the problem of unbalanced and insufficient development is serious, and the per capita income level lags behind that of high-income countries. Therefore, we cannot directly learn from the identification criteria in high-income countries; after the absolute poverty standard is changed to the relative poverty standard, to alleviate its impact on the poverty alleviation policy, it is reasonable to use the median income of 40% as the relative poverty standard (Li X. H. et al., 2020). Considering China’s actual and international experience, this paper selects the median per capita income of 40% of households as the income poverty line of the economic dimension. If the per capita income of households is less than the poverty line, it is assigned as 1, which is in the state of poverty, otherwise, it is 0, which indicates non-poverty.
3.2 Health Dimension
Health itself, as a kind of capital, is both a kind of wealth and an investment product (Wang and Liu, 2006). Having a healthy body can bring more social opportunities, and the level of health has a direct impact on individual labor productivity and social labor productivity. Disease itself not only affects income but also reduces the quality of life of patients, leading to poverty, the afflicted having less capital, and fewer development opportunities. In this paper, the self-rated physical health and the number of hospitalizations for illness with better data availability are selected to comprehensively reflect adults’ health. The self-rated health indicator reflects the perceptual evaluation of rural adults, and the number of hospitalizations reflects their health status. If the self-rated body is significantly unhealthy or relatively unhealthy, the value is 1. If it is relatively healthy or significantly healthy, the value is 0. The value for respondents who have been hospitalized due to illness in the past 12 months is 1, otherwise, it is 0.
3.3 Education Dimension
Education plays an obvious role in expanding individual social opportunities and is also an important way to accumulate cultural capital. The higher the level of education, the more likely individuals are to move to the upper strata of society; On the contrary, the lower the level of education, the more likely individuals are to move to the bottom of society or even fall into relative poverty (Thomas, 2021). As an important labor force in the family, the education status of rural adults plays an important role in the choice and development of livelihood strategies for themselves and their families (He et al., 2019). Therefore, it is also a key dimension to identify MRP. The education level reflects the self-development ability of adults. The indicator level below junior middle school is assigned as 1, otherwise, it is 0. The frequency of reading books, newspapers, or magazines in free time can reflect the acquired habit of continuing education. Hence, if the frequency of reading in free time is daily, or several times a week, a month, or a year or less, it is assigned as 0, and for people who never read, they are assigned 1.
3.4 Human Settlements
From 2014 to 2022, the first document annually issued by the Chinese central government mentioned the improvement of the rural residential environment. The climate-related living environment includes sunshine, rainfall, temperature, and air quality. The climatic factors such as rainfall and temperature are exogenous variables, and most are determined by regional climatic conditions. However, the sunshine level of the respondents’ residential houses and the air quality of the self-rated residential environment are determined by climatic factors, local economic development level, and affected by personal decision-making factors such as the owner’s building site, orientation, and floor. It can better reflect personal feelings about the sunshine time of residential houses and the air quality of the living environment. According to the division of China’s climate zones and the number of urban permanent residents, the sunshine time of residential buildings should be more than two or 3 hours on a cold day and more than 1 hour on the winter solstice. Considering that the residential population density in rural areas is much lower than that in cities, and the sunshine time of houses is more abundant, the average in winter is less than 2 hours, which is assigned as one and greater than or equal to 2 hours, which is assigned as 0. Regarding the air quality of the indicator living environment, if the respondents agree or significantly agree that the air quality is good, the value is 0; otherwise, the air quality is poor, and the value is 1. See Table 1 for specific dimensions and indicator selection, critical values, and weights.
4 MRP Measurement Results of Chinese Rural Adults Considering Climate Factors
4.1 Data Sources and Research Methods
This paper adopts CGSS, which covers the survey data from 2003 to 2018, as the main data source for the study of Chinese society. It is widely used in scientific research, teaching, and government decision-making. The sunshine time data was added in 2018. In this paper, the latest data from 2018 were used to obtain a sample of 1,311 adults in rural China after data cleansing. This article will further refine the data into seven areas in rural China, respectively, the Northeast (Heilongjiang Province, Jilin Province, and Liaoning province), East China (Shanghai Municipality, Jiangsu Province, Zhejiang Province, Anhui Province, Fujian Province, Jiangxi Province, Shandong Province, Taiwan province), North China (Beijing, Tianjin Municipality, Shanxi Province, Hebei Province, Inner Mongolia autonomous region), Central China (Henan Province, Hubei Province, Hunan Province), South China (Guangdong Province, Guangxi Zhuang Autonomous Region, Hainan Province, Hong Kong Special Administrative Region, Macao Special Administrative Region), Southwest China (Sichuan Province, Guizhou Province, Yunnan Province, Chongqing Municipality, Tibet Autonomous Region), and Northwest China (Shaanxi Province, Gansu Province, Qinghai Province, Ningxia Hui Autonomous Region, Xinjiang Uygur Autonomous Region). Among them, the database does not have data for Taiwan Province, Hainan Province, Tibet autonomous Region, Xinjiang Uygur Autonomous Region, or the Hong Kong and Macao special administrative regions.
This paper uses the A-F method, which is widely used by the United Nations and other organizations and many scholars to calculate multidimensional poverty, to measure the MRP of rural adults. A-F method includes two Cutoffs, also known as the dual Cutoff Identification Approach. The first step is to set multidimensional poverty indicators and corresponding indicator deprivation critical values. The second step is to calculate the aggregate deprivation score of an individual and set the aggregate deprivation critical value. If the aggregate deprivation score of an individual exceeds this critical value, it is identified as multidimensional poverty (Alkire and Foster, 2011).
Assuming that the rural individuals are composed of
Defines a vector
This paper uses the equal-weighted method, to sum up the deprivation values of various indicators of MRP. Let the weight vector be
If
4.2 Indicators of Poverty Include the Results of Climate Factors
Table 2 shows the indicator poverty rate of rural adults in different regions of China calculated according to the MRP indicator system of rural adults including climate factors. As seen in the overall indicator poverty rate of China in Table 2, the education level in the education dimension indicators and the frequency of reading, newspapers, or magazines in free time show higher poverty rates, both exceeding 39%. The poverty rate of air quality in the dimension of human settlements is 28.07%, ranking third among all seven indicators, but the poverty rate of the housing sunshine level indicator is low, which is 7.70%. Generally, the poverty of air quality indicator is prominent in China, while that of the housing sunshine level indicator is not.
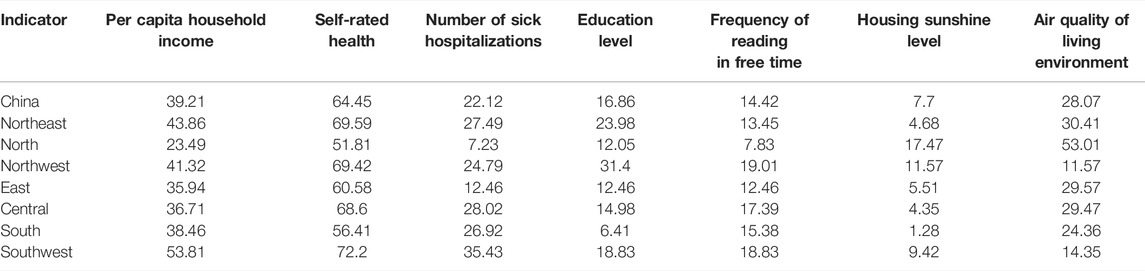
TABLE 2. Overall indicator poverty rate of Chinese rural adults including climate factors (units: %).
North and Northwest regions of housing sunshine level indicator poverty rate are over 11%, while other areas of the housing sunshine level indicator poverty rates are below 9.5%. Northwest and Southwest air quality of living environment indicator poverty rates are less than 14.4%, while in the Central, South, Northeast, and East regions the air quality of living environment indicator poverty rates is between 24% and 30.5%, The poverty rate of air quality of the living environment in the North is 53.01%. Comparing the indicator poverty rates for the Northeast, East, Central, South, Southwest, and Northwest with North China shows that the indicators of air quality and housing sunshine level in North China are the poorest. The housing sunshine level in South China and the air quality in Northwest China are the best. The economy and industry of North China are relatively developed, with severe haze in winter, and its geographical location is in the north of China. Therefore, the poverty rate of housing sunshine level, and air quality indicators in this region are the highest, but that of economic and educational indicators is the lowest. The results show that the MRP including sunshine level and air quality indicators can reveal the regional poverty difference of human settlements under the double superposition of climate and economic factors.
4.3 MRP Index Including Climate Factors
K = 1, 2, or 3 respectively indicate that rural adults have MRP with more than one dimension, two dimensions, or three dimensions. The MRP indicator system has four dimensions, and K can be assigned as 1, 2, or 3. Among them, the K = 1 standard can identify the MRP population with more than one dimension, two dimensions, and three dimensions, and the incidence of MRP under the K = 1 standard is the highest. The K = 2 standard can identify the MRP population with more than two dimensions and more than three dimensions, whose incidence of MRP is less than the MRP rate under the K = 1 standard. The K = 3 standard can identify the MRP population in more than three dimensions, and the incidence of MRP under this criterion is the lowest. Table 3 describes the incidence of poverty, average deprivation degree, and MRPI of rural adults in various regions of China when K = 1, 2, and 3. When K = 1, the MRPI for the 7 regions are ranked from high to low as Southwest, Northeast, Northwest, Central, South, East, and North China. When K = 2, the MRPI indices for the 7 regions from high to low are Northeast, Northwest, Southwest, Central, East, South, and North China. When K = 3, only the Northwest, East, and Southwest regions of MRPI have more than three dimensions of MRP population, while the incidence of MRP in other regions is 0.
Comparing the MRP measurement results of China’s overall, Northeast, East, North, Central, South, Southwest, and Northwest China in Table 3, we find that there are regional differences in China’s MRP situation. North China is the richest in the country, with the lowest MRPI, and the MRP situation in this area is the least serious, while Southwest China and Northeast China have higher MRPI under the standard of K = 1, 2, or 3, and show a relatively serious MRP condition.
4.4 Contribution Rate of MRP Dimension and Indicator Including Climate Factors
Tables 4, 5 further break down the MRP considering climate factors to obtain the contribution rates of different dimensions and indicators of rural adults in China and Northeast, East, North, Central, South, Southwest, and Northwest China regarding MRP. By comparing the measurement results of the contribution rates of different dimensions to MRP in Table 4, it can be seen that the contribution rates of different dimensions in China are education, economic, health, and human settlements in descending order. By region, under K = 1 and K = 2 standards, the contribution rate of human settlements dimension in the North is higher than that of the health dimension, while that of other regions is lower than that of the health dimension. Under the K = 3 standard, the contribution rate of human settlements’ environment and economic dimensions in the North is 0.
Regarding China as a whole in Table 5, when K = 1, 2, or 3, the contribution rate of income indicator is the highest, and that of the housing sunshine level of residential houses and self-rated air quality indicator are low. In terms of regions, when K = 1, for Northeast, Northwest, and Southwest regions, frequency of reading in free time and per capita household income are the indicators with higher contribution rates, while number of sick hospitalizations, housing sunshine level, and air quality of living environment are the indicators with lower contribution rates. For North China, the indicator contribution rate of education level, frequency of reading in free time, and air quality of living environment is higher, while the contribution rate of the number of sick hospitalizations is lower. For East China, the contribution rate of education level, frequency of reading in free time, and per capita household income is higher, while the contribution rate of housing sunshine level is lower. For central China, the contribution rate of frequency of reading in free time and household per capita household income is higher, while the contribution rate of housing sunshine level is lower. For South China, the contribution rates of frequency of reading in free time and per capita household income are higher, while the contribution rates of self-rated health and housing sunshine level are lower.
Regarding regions, different from the other six Chinese regions, the economically developed North China has higher indicator contribution rates of sunshine level and air quality in the dimension of human settlements than the self-rated health and the number of sick hospitalizations in the dimension of health. Similar to the results in Table 2, the characteristics of indicator contribution rate in North China also show that for economically developed regions in Northern China, MRP including sunshine level and air quality indicator can measure the difference in indicator contribution rate between regions under the superposition of climate and economy. This further shows that the sunshine level and self-rated air quality are the indicators to be selected, and their necessity exceeds the self-rated health and the number of sick hospitalizations in the health dimension.
5 Research Conclusion
Relative poverty, which takes climate into account, needs to be measured in multiple dimensions. The multidimensional poverty measurement method has achieved good results in China, and the multidimensional poverty identification and withdrawal criteria of “Two Assurances and Three Guarantees” in Rural China have laid a solid foundation for the MRP identification, measurement, and governance. However, poverty also exists in the dimensions of health, education, and human settlements. In Table 2, the poverty rate of the self-rated health indicator in the health dimension is higher than that of the Per capita Household income indicator in the economic dimension. For the North region, the indicator poverty rate of Air quality of living environment in the dimension of human settlements exceeds that of Per capita household income. Wang and Feng (2020) also conclude that China’s relative poverty needs multidimensional measurement, and suggested that the MRP indicator system should include human settlements factors.
The housing sunshine level and the air quality indicator of living environment are the comprehensive embodiment of people’s micro-climate, economic development, and pollution degree. As shown in Table 2, the indicator poverty rate of housing sunshine level in North China is the highest, 17.47%, and that in South China is the lowest, 1.28%. The poverty rate of air quality of living environment indicator in North China is the highest, 53.01%, and the lowest in Northwest China at 11.57%. Selecting the indicators of the sunshine level and air quality can measure the regional differences of MRP in China under the superposition of economic climate.
According to the results of the MRP measure, the Chinese mainland can be divided into four types. As shown in Figure 1, the horizontal axis represents the regional economic development level and the vertical axis represents the quality of regional human settlements. Region I is an area with a rich economy and human settlements, region II has a poor economy but rich human settlements, region III has a poor economy and poor human settlements, and region IV has a rich economy but poor human settlements. The poverty and contribution rates of economic indicators in North China are low; however, the indicator poverty rate and the contribution rate of the dimension of human settlements are high. Hence, this area is a typical representative of region IV, being economically rich but its human settlements poor. Southwest China is a typical representative of region II with rich human settlements but a poor economy. The indicator poverty rate and the contribution rate of the economic dimension are at a high level, but the indicator poverty rate and the contribution rate of the human settlements dimension are low. The classification of regions will change with the changes in regional economic development level and human settlement. Region Ⅱ (economic development level in poverty but human settlements rich) will change into region Ⅰ (level of economic development and human settlements rich) by developing the local economy, but if the economic development has brought the serious pollution, the region Ⅰ will change into region Ⅳ (economic development level rich but human settlements poor).
The measurement results in North China show that the relevant indicators of climate factors such as housing sunshine level and air quality can identify the poor people with lack of sunshine and poor air quality, and measure the regional MRP difference caused by the superposition of economic climate. The industrialization and economic level of North China are higher than the Chinese mainland, but it also brings haze pollution (Li, 2018). This reveals a typical phenomenon of regional social and economic development: the highly industrialized economic development may be at the expense of the environment, which is a “blind spot” of MRP measure and does not consider sunshine and air factors. This study has made up for this shortcoming. Therefore, identifying and measuring the poverty in the dimension of human settlements will help in studying and formulating targeted governance measures. Some such measures are as follows: Strengthen departmental cooperation and establish climate emergency plans for joint prevention and controlling the meteorological department. Set afforestation protection areas to prevent over-exploitation of forest and other green areas. Drawing lessons from the building standards of urban houses, setting construction standards for rural houses in terms of climate such as sunshine hours and rectifying a number of rural houses that lack sunshine. Improve and upgrade the energy use structure in rural areas and promote the use of clean and efficient energy. Improve the environmental protection awareness of rural people, and include them in the knowledge system of compulsory education. The typicality of North China has certain enlightenment for China and other economies in the world to realize the harmonious development of the economy and environment. Only by considering the poverty governance of regional economic development and human settlements, we can comprehensively realize the high-quality life pursued by rural residents.
Data Availability Statement
The original contributions presented in the study are included in the article/Supplementary Material, further inquiries can be directed to the corresponding authors.
Author Contributions
XH conceived the idea for this study. XH conducted the statistical analysis. HW and WW contributed to the final write-up.
Funding
This research was funded by The National Social Science Fund of China (No. 19ZDA151).
Conflict of Interest
The authors declare that the research was conducted in the absence of any commercial or financial relationships that could be construed as a potential conflict of interest.
Publisher’s Note
All claims expressed in this article are solely those of the authors and do not necessarily represent those of their affiliated organizations, or those of the publisher, the editors and the reviewers. Any product that may be evaluated in this article, or claim that may be made by its manufacturer, is not guaranteed or endorsed by the publisher.
Acknowledgments
The valuable comments and suggestions of editors and reviewers are gratefully acknowledged.
References
Ahmed, S. A., Diffenbaugh, N. S., Hertel, T. W., Lobell, D. B., Ramankutty, N., Rios, A. R., et al. (2011). Climate Volatility and Poverty Vulnerability in Tanzania. Glob. Environ. change 21, 46–55. doi:10.1016/j.gloenvcha.2010.10.003
Alkire, S., and Apablaza, M. (2016). Multidimensional Poverty in Europe 2006–2012: Illustrating a Methodology. Oxf. Poverty Hum. Dev. Initiative Work. Pap. doi:10.13140/RG.2.1.3445.8481
Alkire, S., and Foster, J. (2011). Understandings and Misunderstandings of Multidimensional Poverty Measurement. J. Econ. Inequal. 9, 289–314. doi:10.1007/s10888-011-9181-4
Arouri, M., Nguyen, C., and Youssef, A. B. (2015). Natural Disasters, Household Welfare, and Resilience: Evidence from Rural Vietnam. World Dev. 70, 59–77. doi:10.1016/j.worlddev.2014.12.017
Barbier, E. B., and Hochard, J. P. (2018). The Impacts of Climate Change on the Poor in Disadvantaged Regions. Rev. Environ. Econ. Policy 12, 26–47. doi:10.1093/reep/rex023
Burke, M., Hsiang, S. M., and Miguel, E. (2015). Global Non-linear Effect of Temperature on Economic Production. Nature 527, 235–239. doi:10.1038/nature15725
Cattaneo, C., and Peri, G. (2016). The Migration Response to Increasing Temperatures. J. Dev. Econ. 122, 127–146. doi:10.1016/j.jdeveco.2016.05.004
Chen, L. Q. (2020). Gender Differences in Rural Household Poverty and the Multidimensional Poverty Index: An Analysis Based on the Data Collected from Limushan Town in Hainan Province. J. Nanjing Normal Univ. Sci. Ed. 2, 107–115. doi:10.1080/12259276.2017.1279886
Chen, Y. F., Wang, Y. H., Zhao, W. J., Hu, Z. W., and Duan, F. Z. (2017). Contributing Factors and Classification of Poor Villages in China. Acta Geogr. Sin. 72, 1827–1844. doi:10.11821/dlxb201710008
Cheng, M. W., Gai, Q. E., Jin, Y. H., and Shi, Q. H. (2016). Focusing on Human Capital Improvement and Income Growth. Econ. Res. J. 51, 168–181.
Ding, J. J. (2014). Theoretical Basis,Measurement Methods and Practice Progress of Multidimensional Poverty. West Forum 24, 61–70. doi:10.3969/j.issn.1674-8131.2014.01.008
Duan, L. L., and Wang, L. M. (2020). Is the Protection of Green Water and Green Hills Conducive to Regional Anti-poverty-new Evidence from Poor Areas along the Yangtze River Economic Belt. J. Guizhou Univ. finance Econ. 4, 78–88. doi:10.3969/j.issn.1003-6636.2020.04.009
Gustafsson, B., and Li, S. (2004). Expenditures on Education and Health Care and Poverty in Rural China. China Econ. Rev. 15, 292–301. doi:10.1016/j.chieco.2003.07.004
Haines, A., and Ebi, K. (2019). The Imperative for Climate Action to Protect Health. N. Engl. J. Med. 380, 263–273. doi:10.1056/NEJMra1807873
Hallegatte, S., and Rozenberg, J. (2017). Climate Change through a Poverty Lens. Nat. Clim. Change 7, 250–256. doi:10.1038/nclimate3253
He, R., Fang, F., and Liu, Y. (2019). Influence of Human Capital on the Livelihood Strategy of Farming Households in Poor Mountainous Areas: A Case Study of Liangshan Yi Autonomous Prefecture of Sichuan, China. Prog. Geogr. 38, 1282–1893. doi:10.18306/dlkxjz.2019.09.002
He, R. W., Li, G. Q., Liu, Y. W., Li, L. N., and Fang, F. (2017). Theoretical Analysis and Case Study on Targeted Poverty Alleviation Based on Sustainable Livelihoods Framework: A Case Study of Liangshan Yi Autonomous Prefecture, Sichuan Province. Prog. Geogr. 36, 182–192.
He, R. W. (2018). Research Progress on the Formation Mechanism of Rural Poverty in China and the Construction of Poverty Research Framework. Guangxi Soc. Sci. 7, 166–176. doi:10.3969/j.issn.1004-6917.2018.07.032
Huang, Y., Liu, Y., and Wu, H. (2016). The Finance-Growth Nexus and Poverty Reduction in Western China. Emerg. Mark. Finance Trade 52, 513–521. doi:10.1080/1540496x.2016.1110469
JRF (2020). UK Poverty 2019/2020 Report. Avaliable at: https://www.Jrf.org.uk/report/uk-poverty-2019-20 (Accessed February 7, 2020).
Leichenko, R., and Silva, J. A. (2014). Climate Change and Poverty: Vulnerability, Impacts, and Alleviation Strategies. WIREs Clim. Change 5, 539–556. doi:10.1002/wcc.287
Li, S., Li, Y. Q., and Li, Q. H. (2020a). From Absolute Poverty to Relative Poverty: The Dynamic Evolution of Rural Poverty in China. J. S. China Norm. Univ. Soc. Sci. Ed. 6, 30–42.
Li, X. H., Zhou, Y., and Chen, Y. F. (2020b). Theory and Measurement of Regional Multidimensional Poverty. Acta Geogr. Sin. 75, 753–768. doi:10.11821/dlxb20200400-7
Li, X. L. (2018). Study on the Causes and Control of Haze in North China. Environ. Dev. 30, 74–75. doi:10.16647/j.cnki.cn15-1369/X.2018.05.045
Li, Y., Yu, X. T., and Li, F. (2021). The Standard Definition and Scale Measurement of China’s Relative Poverty. Chin. Rural. Econ. 1, 31–48.
Liu, W., and Wang, X. H. (2020). Stability of Land Ownership and Multidimensional Relative Poverty of Farmers: Ways and Mechanisms of Mitigation. J. Shanxi Univ. Finance Econ. 42, 15–29. doi:10.13781/j.cnki.1007-9556.2020.12.002
Luo, N. S., and Li, J. M. (2018). Do industrial Agglomeration and Traffic Links Aggravate the Spatial Spillover Effects of Haze? Analysis from the Perspective of Spatial Distribution of Industries. Ind. Econ. Res. 4, 52–64. doi:10.13269/j.cnki.ier.2018.04.005
Marotzke, J., Semmann, D., and Milinski, M. (2020). The Economic Interaction between Climate Change Mitigation, Climate Migration and Poverty. Nat. Clim. Chang. 10, 518–525. doi:10.1038/s41558-020-0783-3
OPHI (2020). Global Multidimensional Poverty Index 2020-charting Pathways Out of Multidimensional Poverty: Achieving the SDGs. Report. United Nations Development Programme (UNDP) and Oxford Poverty and Human Development Initiative OPHI. https://hdr.undp.org/sites/default/files/2020_mpi_report_en.pdf
Pan, W. X., and Yan, X. Q. (2020). A Prospective Study on the Establishment of New Rural Poverty Standard after Year 2020. Issues Agric. Econ. 5, 17–27. doi:10.13246/j.cnki.iae.2020.05.002
Pérez-Cirera, V., López-Corona, O., Carrera, G. T. F., Reyes, M., and Garcia-Teruel, A. (2017). Incorporating the Environmental Dimension into Multidimensional Poverty Measurement: An Initial Proposition. ArXiv Preprint ArXiv:1701.04530.
Shen, Y. Y., and Li, S. (2020). How to Determine the Standards of Relative Poverty after 2020?—With Discussion on the Feasibility of “Urban–rural Coordination”. J. South China Normal Univ. 9, 91–101.
Thomas, H. (2021). The Effect of Education on Poverty: a European Perspective. Econ. Educ. Rev. 83, 102124. doi:10.1016/J.ECONEDU-REV.2021.102124
Tigchelaar, M., Battisti, D. S., Naylor, R. L., and Ray, D. K. (2018). Future Warming Increases Probability of Globally Synchronized Maize Production Shocks. Proc. Natl. Acad. Sci. U.S.A. 115, 6644–6649. doi:10.1073/pnas.1718031-11510.1073/pnas.1718031115
Torabi Kahlan, P., Navvabpour, H., and Bidarbakht Nia, A. (2021). Missing Aspects of Poverty: The Case of Multidimensional Poverty in Iran. J. Poverty 1, 14. doi:10.1080/10875549.2021.1925806
Townsend, P. (1979). Poverty in the United Kingdom: A Survey of Household Resources and Standards of Living. Berkeley: University of California Press.
Wang, Q., and Liu, S. (2020). Multidimensional Poverty and Inequality of Migrant Workers in Urban Areas. J. Quantitative Tech. 37, 83–101. doi:10.13653/j.cnki.jqte.2020.01.005
Wang, S. G., and Liu, M. Y. (2019). Implementation Dilemma and Policy Choice of Health Poverty Alleviation. J. Xinjiang Normal Univ. (Philosophy Soc. Sci. 40, 82–91. doi:10.14100/j.cnki.65-1039/g4.20181204.001
Wang, S. G., and Sun, J. N. (2021). China’s Relative Poverty Standards, Measurement and Targeting after the Completion of Building a Moderately Prosperous Society in an All-Round Way: An Analysis Based on Data from China Urban and Rural Household Survey in 2018. Chin. Rural. Econ. 3, 2–23.
Wang, X. L., and Feng, H. X. (2020). China’s Multidimensional Relative Poverty Standards in the Post-2020 Era: International Experience and Policy Orientation. Chin. Rural. Econ. 3, 2–21.
Wang, X. W., and Liu, L. H. (2006). Theoretical Analysis of Becker and Grossman's Health Demand Model. Chin. Health Econ. 279, 28–35. doi:10.3969/j.issn.1003-0743.2006.05.009
Wei, F. Q., and Zhang, H. l. (2021). Measurement on Multidimensional Relative Poverty in Rural Area of China and its Spatial-Temporal Differentiation Characteristics. Statistics Decis. 27, 104–114. doi:10.13546/j.cnki.tjyjc.2021.16.003
Wu, H. T., Hou, Y., and Ceng, Y. F. (2013). Gender Poverty Measurement of Rural Families from the Perspective of Multidimensional Poverty. Stat. Decis. 20, 27–29. doi:10.13546/j.cnki.tjyjc.2013.20.012
Xiong, N., and Song, H. L. (2018). Study on the Population Structure and Location Evolution of Urban Poverty in Post Development Areas. Soc. Sci. Guangxi 273, 41–44. doi:10.3969/j.issn.1004-6917.2018.03.008
Xu, L. D., Deng, X. Z., Jiang, Q. O., and Ma, F. K. (2021). Identification and Poverty Alleviation Pathways of Multidimensional Poverty and Relative Poverty at County Level in China. Acta Geogr. Sin. 76, 1455–1470. doi:10.11821/dlxb202106010
Yang, F., and Zhuang, T. H. (2021). Construction and Empirical Measurement of Multidimensional Relative Poverty Identification Index System for the New Generation of Migrant Workers from the Perspective of Feasible Ability. West. China 6, 1–14.
Yin, H. D., Wang, Y., and Wang, S. G. (2017). Identification of Poverty Alleviation Relocation Households: Multidimensional Poverty Measurement and its Decomposition. China Population, Resources and Environment China Population. Resour. Environ. 27, 104–114. doi:10.12062/cpre.20170517
Zhang, H., Xu, Z., Wu, K., Zhou, D., and Wei, G. (2019). Multi-dimensional Poverty Measurement for Photovoltaic Poverty Alleviation Areas: Evidence from Pilot Counties in China. J. Clean. Prod. 241, 118382. doi:10.1016/j.jclepro.2019.118382
Zhang, Q. (2020). Measurement and Causes of Multidimensional Poverty of Female Recipients of Subsistence Allowances. J. Shandong Women's Univ. 151, 32–40.
Zhang, Y., and Duan, W. (2020). Challenges of Relative Poverty Research and Governance and its Enlightenment to China. Soc. Sci. Abroad. 6, 57–65.
Zhang, Y. H., and Jin, L. S. (2006). A Case Study of Linkage between Environment and Poverty in the Semi Arid Northwestern China. J. China Agric. Univ. Soc. Sci. Ed. 1, 8–13. doi:10.13240/j.cnki.caujsse.2006.01.003
Zhong, C., and Lin, M. (2020). A Study of Multidimensional Deprivation and Influencing Factors of Relatively Poor Families in. Chin. J. Nanjing Agric. Univ. Soc. Sci. Ed. 20, 112–120. doi:10.19714/j.cnki.1671-7465.2020.0062
Keywords: multidimensional relative poverty, sunshine time, rural China, climatic factors, regional differences
Citation: Hou X, Wu H and Wang W (2022) Identification and Measurement of Multidimensional Relative Poverty of Chinese Rural Adults Considering Climate Factors. Front. Environ. Sci. 10:891077. doi: 10.3389/fenvs.2022.891077
Received: 07 March 2022; Accepted: 05 April 2022;
Published: 12 May 2022.
Edited by:
Shaoquan Liu, Institute of Mountain Hazards and Environment (CAS), ChinaReviewed by:
Guanglin Sun, Nanjing University of Finance and Economics, ChinaGuo ChangQing, Lanzhou University of Technology, China
Yibin Ao, Chengdu University of Technology, China
Copyright © 2022 Hou, Wu and Wang. This is an open-access article distributed under the terms of the Creative Commons Attribution License (CC BY). The use, distribution or reproduction in other forums is permitted, provided the original author(s) and the copyright owner(s) are credited and that the original publication in this journal is cited, in accordance with accepted academic practice. No use, distribution or reproduction is permitted which does not comply with these terms.
*Correspondence: Xiaoyuan Hou, houxiaoyuan_hxy@126.com; Haitao Wu, wuhan_haitao@aliyun.com