Abstract
Climate change is expected to impact the habitability of many places around the world in significant and unprecedented ways in the coming decades. While previous studies have provided estimates of populations potentially exposed to various climate impacts, little work has been done to assess the number of people that may actually be displaced or where they will choose to go. Here we modify a diffusion-based model of human mobility in combination with population, geographic, and climatic data to estimate the sources, destinations, and flux of potential migrants as driven by sea level rise (SLR) in Bangladesh in the years 2050 and 2100. Using only maps of population and elevation, we predict that 0.9 million people (by year 2050) to 2.1 million people (by year 2100) could be displaced by direct inundation and that almost all of this movement will occur locally within the southern half of the country. We also find that destination locations should anticipate substantial additional demands on jobs (594 000), housing (197 000), and food (783 × 109 calories) by mid-century as a result of those displaced by SLR. By linking the sources of migrants displaced by SLR with their likely destinations, we demonstrate an effective approach for predicting climate-driven migrant flows, especially in data-limited settings.
Export citation and abstract BibTeX RIS

Original content from this work may be used under the terms of the Creative Commons Attribution 3.0 licence.
Any further distribution of this work must maintain attribution to the author(s) and the title of the work, journal citation and DOI.
Introduction
Migration is an important adaptive strategy for coping with environmental and climate changes. An individual's decision to migrate depends on a host of factors from livelihood opportunities to household assets, social connections and perceived risk [1, 2], yet there is little understanding of how all of these economic, political, social, and demographic considerations ultimately influence adaptive behavior, particularly in the face of environmental risk and climate variability (e.g. [3]). A wealth of recent work has attempted to disentangle this complexity by assessing the environmental and climatic drivers of human mobility and migration in developing countries and made strides to predict if and how these dynamics may change in the future [4]. Numerous studies using empirical models have shown that climate variability significantly influences migration in Indonesia [5], Mexico [6], South Africa [7], Bangladesh [8], Pakistan [9] and many other countries (e.g. [10, 11]). New applications of agent-based modeling (ABM) to estimate environmental migration also represent a promising development in capturing individual actions and decision-making (e.g. [12–16]). These and many other studies have been instrumental in exploring different aspects of the migration-climate relationship (permanent vs. temporary; internal vs. international; climate-driven vs. disaster-driven). However, almost all of these approaches have been data-intensive (either for parameterization, validation, or extrapolation), geographically specific, and/or are based on historical statistical relationships between changes in climate and human migration—the dynamics of which may well change with time [17]. These qualities likely limit the utility of such approaches across a variety of settings, particularly in places where information is sparse. It is therefore essential to develop new and reliable methods that can—with minimal inputs—be applied in a range of situations to predict migration flows as a result of climate variability and that can be used as a basis for informing adaptive strategies [18, 19]. Here we modify an existing parameter-free model to investigate migrations resulting from the emergence of environmental conditions that render a place uninhabitable or less suitable for human activities (e.g. loss of livelihoods, damage or destruction of assets). We use the example of sea level rise (SLR) in Bangladesh—a densely populated country with a rural economy—to explore the efficacy of this alternative, stochastic modeling approach in estimating migrant sources, destinations, and flux and discuss its potential application to a host of climatic drivers of migration.
SLR is expected to have profound effects on livelihoods and food security in the coming decades [20]. From physical inundation to saltwater intrusion to higher-intensity storms, SLR poses a serious adaptation challenge for coastal human and natural systems. The spectrum of vulnerability, exposure, and response to SLR will be dictated by a variety of factors including geophysical properties of coastlines and storms, adaptive capacity of governments, and socio-economic status of coastal communities [21, 22]. Certain countries (e.g. [23]) and cities (e.g. [24])—whose coastal populations continue to increase despite being substantially exposed to the impacts of flooding and SLR [25, 26]—will likely adopt adaptation steps [27], while many developing nations possess limited means for protective measures (e.g. sea walls, dikes, floodgates, canals, and pumps) [28]. Thus, for vulnerable populations living in these low-lying coastal regions, migration will continue to be an important adaptation strategy [1, 2, 21, 28].
Numerous recent studies (e.g. [23, 28–32]) have assessed the exposure of future populations to SLR and coastal flooding. However, how and to what extent the effects of SLR will influence the movements of people—as well as the specific sources and destinations of these potential migrants—remains poorly understood. This is especially true for Bangladesh, a country with 41% of its population (53 million people) living in low elevation (<10 m) coastal zones [32], regularly exposed to a host of natural extreme events, and with a long history of adaptation and flood management efforts [33]. For instance, in the aftermath of cyclone Aila in 2009, lack of access to food and shelter led to the substantial migration of coastal residents (e.g. [34, 35]) with only some able to return to their homes a few months later [36]. Unlike these types of abrupt and relatively short-lived natural hazards (e.g. floods, cyclones) that have induced widespread displacements in Bangladesh [8, 37], SLR represents a different type of migration driver, in that residents will not have the option to return to their origin after a certain period of time. Indeed, a recent survey of migrants to Dhaka and Khulna in 2010 showed SLR and its associated livelihood impacts were already influencing movements within the country [34].
By combining information on population distribution, elevation, and climate change scenarios within a parameter-free model of human mobility (via generalization of the so-called 'radiation' model [38] to account for SLR), we estimate the cumulative sources, destinations, and number of people displaced by direct inundation alone due to future mean SLR in Bangladesh for the years 2050 and 2100. While the benefits of adaptation in reducing vulnerability to flooding are well known (e.g. [26]), it is unclear what Bangladesh's efforts at flood management have meant for prevented environmentally driven migration, and it is uncertain where and to what extent further adaptation steps will be implemented in the coming decades (though many plans are in various stages of realization (e.g. Bangladesh Delta Plan 2100)). Because of these data limitations linking migration and adaptation in the country, we assume no further adaptation to sea level rise in the SLR scenarios that we consider. Our results are validated using government data on inter-provincial migration in Bangladesh, and then used to predict future migration patterns, and the consequent impacts on jobs, housing, and food security. In doing so, we aim to address a substantial gap recently identified in the environment-migration literature [39] by providing a universal, minimum data model as a basis for the study and comparison of future migration scenarios as influenced by climate change.
Materials and methods
Inundation simulation under sea level rise (SLR) scenarios
Elevation data were taken from the Shuttle Radar Topography Mission (SRTM Global 1 arc second Version 3.0) for Bangladesh [40]. These digital maps provide integer-value elevations at resolution up to 1 arc second (∼30 meters) and capture large embankments (polders) that are currently in widespread use throughout Bangladesh to protect against rising seas. These data were resampled to a 90 m per pixel resolution (by averaging every 3 by-3 9 pixel block) to estimate elevation values to tenths of meters. Because of the absence of high precision DEM (digital elevation model) data for Bangladesh, numerous previous studies have used SRTM data (e.g. [41, 42]) in a similar way, keeping in mind the limitations on vertical height accuracy of such datasets. We then used the eight-side connectivity rule for inundation modeling whereby the locally protected low elevation grid cell will get inundated if its elevation is less than the projected sea level and if one of its eight neighbors was either a flooded pixel or open water (see [43]).
To simulate future mean SLR impacts on coastal population in Bangladesh, we used SLR projections for four Representative Concentration Pathway (RCP) scenarios from the IPCC's Fifth Assessment Report [20]. For the year 2050, we only used the RCP 8.5 SLR projection (corresponding to 8.5 W m−2 increase in radiative forcing with respect to pre-industrial levels) of 0.30 m as the other three pathways all had similar SLR projections. For the year 2100, we used projected global mean SLR for four RCP pathways—namely, RCP 2.6, RCP 4.5, RCP 6.0, and RCP 8.5—which correspond to a projected SLR of 0.44, 0.53, 0.55 and 0.74 meters, respectively. We also modeled inundation for mean SLR projections of 1.5 and 2 meters as a representation of more rapid SLR scenarios in 2100. These values are in line with the upper range of SLR utilized in numerous recent studies (e.g. 2.0 m [44], 2.25 m [45], and 2.4 m [46]). Further, because our analysis considers mean SLR without normal high tides, this helps to ensure that our estimates of inundated area—and displaced population—are conservative. In addition, because we perform inundation modeling to ensure that flooded pixels have connectivity with the ocean, our estimates of inundated areas are also conservative relative to previous studies (e.g. refs. [29, 47, 48]).
Demographic projections
District-(zila-)level population for the year 2010 was estimated by aggregating the Gridded Population of the World (GPWv4) dataset [49] (30 arcsecond grid) and adjusting to UN medium-variant population estimates. Separately, we calculated Ti,2010—the population in the year 2010 that would be inundated in district i—for each of the 64 zilas (districts) by overlaying each inundation map with GPWv4 data and summing the GPWv4 grid cells contained within the inundated area. This value was then projected for the years 2050 and 2100 as:
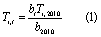
where b2010 is the year 2010 population of Bangladesh and bt is the medium-variant projected population of Bangladesh for either the year 2050 or 2100 [50].
Baseline radiation model
The 'radiation' model [38] is an alternative approach to gravitational models [51] for the simulation of migration fluxes between cities. In this model the term 'radiation' does not refer to the radiative forcing of the climate change scenarios but to a statistical mechanics approach to the modeling of migration that resembles the physics of radiation [38]. The main advantage of this model is that it is parameter free. It is derived starting at the microscopic scale and upscaling human migrations using a statistical mechanics approach. To make this study more self-contained, we first briefly review the model by Simini et al [38], and then explain how the model was modified to account for the effect of SLR.
The baseline model is based on the following steps:
-
1.Every individual, X, leaving from location i is associated with a positive number, zX(i), representing the absorption threshold for that individual. zX(i) is generated as the maximum number obtained after mi random extractions from a pre-selected distribution, p(z), with mi being the population in i. Thus, on average, individuals leaving from a highly populated region have a higher absorption threshold than those emitted from a scarcely populated location. Simini et al [38] have shown that the particular choice of p(z) does not affect the final results.
-
2.The surrounding cities have a certain probability to 'absorb' individual X: zX(j) represents the absorbance of city/region j for individual X, and it is the maximum of nj (the population in location j) extractions from p(z). The individual stops in the closest location that has an absorbance, zX(j), greater than its absorption threshold, zX(i).
By repeating these steps for a given number of outgoing individuals (in our case the predicted emigrants), the model calculates the fluxes among the different locations. The model thus gives an analytical expression for the probability that an individual moving from a location i with population mi will not stop in the closest locations and will arrive in the next location with population nj. This is formally expressed as:
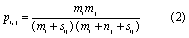
where sij is the total population (within the circle of radius rij centered at i) minus mi and nj. rij is the distance between i and j. Baseline model results showed good agreement (logarithmic regression fits: Lifetime migrants −R2 = 0.75; 5 year migrants −R2 = 0.69) with available division-level internal migration data from the 2011 Bangladesh Census [52] (figure 1).
Figure 1. Division-level comparison with internal migration data. Values represent the log-transformed percentage of total migrants (log(x + 1)), and each point represent a source-destination link (e.g. those who were born in Barisal division and migrated to Dhaka division).
Download figure:
Standard image High-resolution imageRadiation model with sea level rise
To simulate migrations among districts of Bangladesh that are each affected differently by SLR, we modify the radiation model by Simini et al to account for the fact that migration fluxes toward inundated areas are less likely to happen. Following the original model, we denote the population of the source district as mi and the population of the destination district as nj (index i will thus refers to the source, while index j to the destination). Then, following the same modeling steps presented above, we write the probability that a migrant from a region i moves to region j, as:
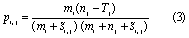
where Tj is the total number of people that are expected to migrate from district j because of inundation and depends on the susceptibility to inundation of district j; is the population living within an area of radius rij (i.e. the distance between points i and j) centered at i. Distances were calculated based on the centroid of each district.
The difference with respect to the classic radiation model (equation 2) is that nj has been replaced with nj − Tj and sij with to account for the fact that if a district j is more vulnerable to inundation (and thus has a high Tj) it will be less likely to be chosen as a destination by migrants and therefore—for the purposes of the migration analysis—it will be as if its population has dropped to nj—Tj.
The average flux from district i to district j can then be calculated as:

and its variance as

Migration fluxes between pairs of districts, i and j, are calculated using equations (3) and (4) with mi and nj determined based on population data and projections based on demographic data for Bangladesh, while Tj is estimated as the population of district j that will be affected by the inundation under the various SLR scenarios considered in this study.
Migrant support at destinations
Average calorie consumption for the year 2010–2439 kcal cap−1 day−1—was taken from the FAO's Food Balances Sheets [53]. Average household size −4.48 people per household—was estimated as the ratio between population and number of households [52]. Dependency ratio (i.e. the ratio of those employed to the total population) (0.675) was taken from the World Bank's World Development Indicators database [54]. Each of these factors was then multiplied by the number of arriving migrants driven by SLR to estimate the additional food, housing, and jobs required at migrant destinations.
Figure 2. Inundation of mean sea level rise scenarios for Bangladesh. Scenarios presented in the map represent the smallest (RCP 8.5; year 2050) and largest (2.0 m) areas inundated amongst the scenarios of this study. The areas inundated under the other scenarios correspond by and large to that of RCP 8.5 year 2050. Population density map is for the year 2010.
Download figure:
Standard image High-resolution imageLimitations and caveats
This work presents a stochastic model to study human migration in Bangladesh under different SLR inundation scenarios. There are several factors would need to be fully considered before comprehensively assessing SLR impacts on the coastal populations of Bangladesh. First, we do not attempt to temporally disaggregate migrant flows. The timing, delay, or prevention of such flows can, on one hand, be influenced by adaptive measures taken by a household [55] or government [56] and, on the other hand, will likely occur in pulses—with some return migration—as a result of increased exposure to flooding events before eventual inundation [23, 57, 58]. Second, we assume no return migration of the migrants displaced by SLR. Because there are a greater number of economic opportunities and the rural-to-urban migrants are diversifying their livelihoods—often adopting occupations that are not dependent on natural resources [16] and with improved access to education and health services—these future migrants are expected to continue living in urban areas (e.g. [59]). As the migrants from the southern region of Bangladesh are disproportionately influenced by environmental factors [59], the degradation of their land caused by SLR (and other associated impacts such as saltwater intrusion and storm surge) will likely further deter urban-rural migrants. Third, we utilize scenarios of global mean SLR and of national average population growth, both of which in reality will occur with greater spatial heterogeneity [60, 61]. Fourth, we do not account for potential future adaptation measures and livelihood opportunities which are perhaps impossible to incorporate beyond the time frame of most planning policies. Nonetheless, the extent to which these measures can be accounted for can have significant impacts on the magnitude, origins, and destination choices of migrants. Fifth, the model is calibrated based on current migration patterns and decisions, and the relative importance of the factors influencing migration may change under future climate. Overall, the bulk of these assumptions ensure that our estimates of migrant flows are conservative.
Table 1. List of the most affected zilas under selected SLR scenarios. Zilas are ordered based on population displaced under RCP 8.5 (year 2050). Values represent the population displaced and area inundated, and percentages represent the proportions of each zila's population and area that would be inundated. Results for all zilas are reported in table S6.
District | State | RCP 8.5 2050 (0.30 m) | RCP 4.5 2100 (0.53 m) | 1.5 m 2100 | |||||||||
---|---|---|---|---|---|---|---|---|---|---|---|---|---|
Popul. | % | km2 | % | Popul. | % | km2 | % | Popul. | % | km2 | % | ||
Shariatpur | Dhaka | 114642 | 6.9 | 208 | 16.0 | 95157 | 6.9 | 208 | 16.0 | 91321 | 6.6 | 222 | 17.1 |
Naray Angonj | Dhaka | 112135 | 2.6 | 37 | 4.7 | 91972 | 2.6 | 37 | 4.7 | 128839 | 3.6 | 47 | 6.1 |
Munshigonj | Dhaka | 108092 | 5.2 | 109 | 11.7 | 90627 | 5.2 | 109 | 11.7 | 82475 | 4.7 | 126 | 13.5 |
Barisal | Barisal | 105879 | 3.3 | 73 | 3.3 | 88765 | 3.3 | 74 | 3.3 | 150138 | 5.5 | 144 | 6.4 |
Chandpur | Chittagong | 78402 | 2.3 | 114 | 7.4 | 63006 | 2.2 | 114 | 7.4 | 67104 | 2.3 | 117 | 7.6 |
Khulna | Khulna | 60959 | 1.9 | 32 | 0.9 | 51111 | 1.9 | 32 | 0.9 | 142968 | 5.2 | 89 | 2.5 |
Comilla | Chittagong | 44688 | 0.6 | 25 | 0.8 | 36274 | 0.6 | 26 | 0.8 | 24695 | 0.4 | 26 | 0.8 |
Shatkhira | Khulna | 37061 | 1.3 | 81 | 2.4 | 31074 | 1.3 | 81 | 2.4 | 108361 | 4.7 | 195 | 5.8 |
Pirojpur | Barisal | 35572 | 2.3 | 34 | 2.7 | 29825 | 2.3 | 34 | 2.8 | 27388 | 2.1 | 38 | 3.1 |
Cox's Bazar | Chittagong | 27781 | 0.9 | 15 | 0.7 | 23293 | 0.9 | 15 | 0.7 | 89837 | 3.5 | 44 | 2.0 |
% of total affected | 81.9 | ... | 86.5 | ... | 81.8 | ... | 86.5 | ... | 61.6 | ... | 54.2 | ... |
Results
We first applied our model to predict human migration in Bangladesh for the year 2011 without accounting for the effect of SLR. In this way we were able to test our theoretical framework for a case where we have available data on population fluxes among divisions (states). The baseline results of the modified 'radiation' model showed good agreement with state-level internal migration data from the 2011 Bangladesh Census [62] (figure 1), the only comprehensive bilateral internal migration data available for Bangladesh. This agreement was true when considering either 'lifetime' migrants or migrants who had only relocated within five years of the census. Thus we were able to successfully replicate internal migration in Bangladesh only using information on population distribution and distance.
We then considered SLR projections based on four RCP scenarios from the IPCC's Fifth Assessment Report [20]. We also examined more extreme conditions with a mean SLR of 1.5 and 2.0 meters to investigate more rapid SLR by 2100 (see supplementary materials available at stacks.iop.org/ERL/13/064030/mmedia). We did not consider higher levels of mean SLR, normal high tides, or storm surges as either the likelihood of their occurrence or the long-term response of inhabitants to their effects remains unclear. We found that mean SLR will induce displacements in 33% of Bangladesh's districts under the considered RCP scenarios and 53% under the more intensive conditions (figure 2). By mid-century, we estimate that nearly 900 000 people are likely to migrate as a result of direct inundation from mean SLR alone (table 1). Under the most conservative and extreme scenarios that we consider (0.44 meter and 2 meter mean SLR, respectively), the number of estimated migrants driven by direct inundation could range from 731 000 people to as many as 2.1 million people by the year 2100. For all RCP scenarios, only five districts (or 'zilas')—Barisal, Chandpur, Munshigonj, Naray Angonj, and Shariatpur—act as the source for 59% of all migrants (figure S1). For Shariatpur and Munshigonj in particular, inundation by SLR will also constitute substantial proportions of these districts' areas (16% and 12%, respectively).
In large part because of the generally high population density across Bangladesh, SLR migrants will likely not search far for an attractive destination (see figure 3). Indeed, the chosen destination tends to reflect a trade-off between the employment opportunities that the destination district can give (for which population serves as a proxy), its distance from the migrant's origin, and its own exposure to SLR impacts. Migrant destinations are distributed diffusely across the southern half of the country with certain districts consistently emerging as migrant destinations (e.g. Bagerhat, Dhaka, Jhalakhali, and Madaripur) (figure 4; tables S1–S4). Preferred migrant destinations are consistent across the different SLR scenarios though the magnitude of the flows can vary substantially. Interestingly, though Dhaka is projected to be the top destination across all scenarios, the number of migrants choosing to move to the city remains relatively consistent despite large changes across scenarios in the number of displaced people (table 2). Further, because the population of Bangladesh is projected—under the medium variant population scenario—to undergo a decrease between mid-century and the year 2100, some scenarios of SLR at century's end show fewer people displaced as compared to the RCP 8.5 scenario for 2050 (table 1).
Figure 3. Predicted migrant destinations for selected districts. The Ti values represent the total number of people displaced from the source district.
Download figure:
Standard image High-resolution imageFigure 4. Net migration under sea level rise. Maps show the difference between arriving migrants and departing migrants.
Download figure:
Standard image High-resolution imageWe also estimate the additional jobs, housing, and food that would be required to support these migrants at their destinations (table 2). To sufficiently accommodate the relocation of all of those estimated to be displaced by inundation in the year 2050 will likely require nearly 600 000 additional jobs, 200 000 residences, and 784 × 109 food calories. For the districts where arriving migrants will constitute a substantial percentage of the existing population (e.g. Jhalakati, Khulna, Madaripur), these additional needs may pose a greater challenge for planners and local governments (table S5). More generally, our findings suggest that SLR will serve to accelerate rates of rural-to-urban migration as displaced people relocate to districts where employment opportunities are more concentrated. Destination choices remain relatively consistent across scenarios, but our results highlight that there is large variation in the number of displaced people depending on the SLR scenario considered. This shows that our approach, which incorporates a suite of scenarios, can be used to adequately capture the full range of possible outcomes in terms of migrant flows.
Discussion
Our study was able to reliably replicate internal permanent migration patterns in Bangladesh (figure 1). By incorporating the effect of SLR into this approach, we were able to assess the sources, destinations, and fluxes of migrants displaced under a number of SLR scenarios. We predict that a large percentage of districts will produce SLR migrants, that these migrants will frequently move to neighboring, less impacted districts, and that preferred destinations will be relatively invariant across SLR scenarios—though the magnitude of predicted migrant fluxes vary widely by 2100 (between 731 000 and 2.1 million total migrants) depending on the SLR scenario (figure 4). This demonstrates that likely migrant destinations can be determined with greater confidence than the actual number of arriving migrants. By knowing the origin and destination of migrants and accounting for the uncertainty in the number of migrants, it is possible to begin tailoring strategies that are more sensitive to cultural and social context, better accommodate the needs of the specific migrant group, and minimize conflict over limited services and resources [63]. This paper provides a universally applicable approach that can be used to assess migrations and future human mobility driven by various climate-related impacts (e.g. SLR, drought, flooding). While previous work has been important for enhancing our understanding of the relationship between climate and permanent migration (e.g. [5, 9]), the parameter-free approach that we present offers increased applicability—especially in data-limited settings—over these empirical techniques.
Of course, the permanence and behavior of a migration decision depends in large part on the persistence and magnitude of the migration driver and the capacity of low-lying areas to adapt. While a greater suite of coping strategies may be available for other coastal hazards (e.g. [64, 65]), physical inundation ultimately necessitates relocation. This is especially true for rural coastal inhabitants who—unlike those living in important port cities (e.g. Khulna) that are more economically motivated to implement large-scale adaptation strategies and flood management [66]—will have limited capacity to remain in place and to cope with rising seas, but may also be less financially equipped to migrate [67]. Indeed, though Bangladesh has a long history of flood protection (e.g. [33, 68]), it is unclear to what extent ongoing or future efforts may be able to minimize the impacts of SLR. Furthermore, flood protection through embankments in tidal channels does not allow water to spread over a larger area and, as a consequence, prevents the penetration of higher water levels further inland to less protected areas [69]. By linking vulnerable coastal populations to potential inland immigration effects, our approach can also help to prioritize interventions in areas of potential emigration and inform likely destination sites about the resources and infrastructure that would be required to support increased immigration (table 2).
Table 2. Jobs, housing, and food required to support additional migrant arrivals by destination for the RCP 8.5 2050 (0.3 m) scenario. Complete results are reported in table S5.
Destination district | Additional migrants | Jobs | Housing | 109 kcal yr−1 |
---|---|---|---|---|
Dhaka | 207374 | 139978 | 46289 | 184.61 |
Madaripur | 83913 | 56641 | 18730 | 74.70 |
Comilla | 65181 | 43997 | 14549 | 58.03 |
Jhalakati | 43456 | 29333 | 9700 | 38.69 |
Chandpur | 40688 | 27465 | 9082 | 36.22 |
Bagerhat | 38681 | 26110 | 8634 | 34.44 |
Khulna | 32811 | 22148 | 7324 | 29.21 |
Munshigonj | 30179 | 20371 | 6736 | 26.87 |
Lakshmipur | 27513 | 18572 | 6141 | 24.49 |
Shariatpur | 26657 | 17993 | 5950 | 23.73 |
Bangladesh | 880422 | 594285 | 196523 | 783.78 |
The displacements we consider will be spread over multiple years and will not be the result of a single, abrupt 'shock'. For instance, Bangladesh has experienced 15 major cyclones resulting in half a million deaths between 1960 and 2007 and is predicted to have over 11 million people exposed to potential storm surge impacts by 2050 [70]. Focusing solely on those permanently displaced by SLR—as opposed to those vulnerable to subsidence, saltwater intrusion, or storm surge—provides a conservative estimate of the people likely to migrate in response to a climate change impact. While those vulnerable to saltwater intrusion, flooding, or storm surge may also be included in such an estimate, their choice of coping strategy in response to their respective impact is less clear and would require in-depth behavioral studies to adequately understand and capture in our model. More generally, estimates of 'climate change migrants' for Bangladesh vary widely in definition, magnitude, and replicability (e.g. [30, 71, 72]), and our estimates demonstrate that an analysis of water body connectivity may be a key constraint that has been missing from previous assessments. Indeed, a population can be defined as 'affected' in numerous ways, and how Bangladesh or any other country identifies all communities vulnerable to and potentially displaced by climate change impacts is a crucial consideration in the application of this model but is beyond the scope of this paper [73].
Future work can seek to enhance the model predictions presented here in a number of ways. This is especially true for the estimation of affected areas and displaced populations. First, a thorough quantification of the heterogeneous potential for adaptation—along coastlines and inland, between rural areas and urban centers, and at the household or government level [55, 56]—must be incorporated. While it is difficult to know what decision-makers will implement even a few years into the future, reasonable approximations of adaptation can help to better constrain migration estimates and can exert significant influence over the sources, magnitude, timing, destination choices of migration from vulnerable areas. In addition, while the focus of this study is on model development, extensions of this work can readily incorporate different socio-economic scenarios to better understand the envelope of likely economic and demographic outcomes (e.g. [60, 61]). Second, future analyses need to account for the various indirect effects of SLR (e.g. saltwater intrusion, groundwater salinization, storm surge frequency, altered flow dynamics in upstream reaches of rivers, etc.) which could add substantially to the number of people forced to move. More broadly, making accurate estimates and predictions of the number of people displaced by different climate drivers (SLR, extreme heat, drought, etc.) will be a critical component for the application of this study's model to other situations [73]. As a combination of factors ultimately dictate the migration decision—and not just a single climate driver as we examine here—it will be important to account for the spectrum of social, political, and economic considerations that all influence the adaptive choices of exposed populations [1, 2]. Third, an intercomparison of model requirements and outputs—between empirical approaches, agent-based methods, and our present work—can enhance understanding of the advantages and shortcomings of each and on potential ways to link these different methodologies.
Conclusion
Migration is one of the most important strategies for adapting to the effects of a changing climate. Yet many aspects of human migration in response to climate change remain poorly understood. We examined the case of Bangladesh, a highly populated country particularly vulnerable to the potential impacts of SLR and climate change. Under projected climate scenarios and impacts, we estimated that by the years 2050 and 2100 as many as 900 000 people and 2.1 million people, respectively, could be forced to migrate as a result of direct inundation from SLR. To assess the relocation of these migrants, we first demonstrated that our model predictions showed good agreement with bilateral census data on internal migration. This indicated that our statistical mechanics approach based on a parameter free diffusion model was able to capture the fundamental aspects of the displacement process. After accounting for the effect of SLR, we were able to predict the likely destinations of migrants and evaluate their additional food, housing, and employment needs (as many as 1.4 million jobs, 470 00 residences, and 1.87 × 1012 food calories by 2100). This approach therefore offers an effective and flexible alternative to empirical and agent-based models typically used to predict climate-driven migrations.
Acknowledgments
K F D, A B, P D, and S S designed the experiment. K F D, A B, and S S performed the analysis. K F D, A B, P D, and S S wrote the paper. K F D was funded by The Nature Conservancy's NatureNet Science fellowship.