Abstract
The impact of climate change on economic growth has been the subject of numerous studies in recent years, with macro-econometric analyses estimating the effect of rising temperatures on gross domestic product (GDP) growth rates at the country-level. However, the distributional impact of warming on inequality and poverty at the micro-level remains relatively unexplored. In this paper, we investigate the relationship between temperature and inequality in South Africa at the national and sub-national level. Our analysis reveals a significant -shaped relationship between temperature and inequality indices, with inequality lowest at moderate temperatures (11 ∘C–18 ∘C) and increasing sharply as temperatures increase. We find that the optimal temperatures are lower for inequality measures than for income levels. This indicates that substantial increases in inequality are expected at higher temperatures compared to growth impacts. This effect is particularly noticeable for the poorer segments of the population, whose productivity and wages decline as temperatures increase, while the impact on the richer segments is less significant due to their greater adaptive capacity. In terms of mechanisms, we find that agricultural households are more likely to experience an increase in inequality due to warming. Our findings suggest that global warming has two adverse effects on hot countries: reducing average growth and increasing inequality. We compare the outcomes of the moderate RCP6.0 scenario to a reference scenario without warming and find that by the end of the century, the Gini coefficient in South Africa is expected to increase by 3–6 points, resulting in a potential welfare loss of approximately 50% when combined with the impact of warming on GDP (which alone can reach up to 43% by 2100 in South Africa). Our findings highlight the importance of investigating the distributional effects of climate change at the micro-level, particularly in low- or middle-income countries where vulnerable populations are more susceptible to its impacts.
Export citation and abstract BibTeX RIS

Original content from this work may be used under the terms of the Creative Commons Attribution 4.0 license. Any further distribution of this work must maintain attribution to the author(s) and the title of the work, journal citation and DOI.
1. Introduction
Climate change impacts on economic outcomes, populations, and demographic groups have been the subject of a growing body of scientific research in recent years (Field and Barros 2014). This paper aims to contribute to this trend by providing evidence from multiple spatial scales on income distribution in South Africa that improves our understanding of the role of climate change in widening the inequality gap.
Increasing temperatures have been shown to impact several demographic, socioeconomic, and public health dimensions (Emmerling and Tavoni 2021). Some notable examples include the impact of climate change on economic growth (Dell et al 2009, 2012, Burke et al 2015b, Newell et al 2021), annual income (Deryugina and Hsiang 2014), labor productivity and supply (Graff Zivin and Neidell 2014, Shayegh et al 2020, Dasgupta et al 2021), human capital (Graff Zivin et al 2018), demography (Casey et al 2019), migration (Desmet and Rossi-Hansberg 2015, Shayegh 2017, Cattaneo et al 2019), food security (Deschênes and Greenstone 2007, Dasgupta and Robinson 2021, 2022), human health (Romanello et al 2022, Dasgupta and Robinson 2023a), and energy consumption (Isaac and Van Vuuren 2009, De Cian and Wing 2019). The general conclusion of these studies seems to suggest that (a) climate change is indeed a determining factor affecting many aspects of human development and (b) the scale and direction of the climate change impacts vary by the geographical and socioeconomic conditions of the affected societies. For example, historical increases in temperatures are shown to substantially reduce economic growth in poor countries through a wide range of impacts from reducing agricultural output (Jones and Thornton 2003) to destabilizing political systems (Burke et al 2015a, Bosetti et al 2020). While most of these empirical assessments are conducted at macroeconomic or aggregate levels, the underlying impact channels work at the individual level. Notably, labor force impacts, migration, food security, health, energy demand, and other impacts affect individuals and households directly. However, such impacts are not distributed equally across different income groups. This might be due to differences in (a) geographical distribution of income groups (poorer households are often live in areas with higher exposure to climate hazards with lower capacity to adapt and respond (Rentschler et al 2022)), or (b) their occupational choice (low-income and low-skilled laborers are often employed in sectors relatively more exposed to heat stress such as agriculture and construction (Shonkoff et al 2011)), or (c) their adaptive capacity (richer households have easier access to (costly) adaptation measures to reduce exposure (Fankhauser 2017)). Therefore, heterogeneity in populations in terms of income along with other dimensions (Emmerling and Tavoni 2021) makes it likely that the distributional impacts of climate change will be differential, and hence affect within-country income distributions.
At a global scale, climate change may also affect inequalities between countries in two ways (Taconet et al 2020): either by causing climate-related impacts that fall more heavily on low-income countries (Formetta and Feyen 2019), or by inflicting higher costs of mitigating climate change through reduced emissions that could slow down the economic catch-up of poor countries (Van den Berg et al 2020). However, evidence shows that the impact of climate damages on inequality seems to be more pronounced than those of mitigation costs and their distribution (Gazzotti et al 2021). Therefore, it is essential to investigate the impacts of temperature change on inequality through its impact on economic growth and productivity. Indeed, across countries, the overall economic productivity seems to exhibit a non-linear relationship with annual mean temperature, peaking at 13.6 ∘C and declining sharply beyond it (Burke et al 2015b). However, the extent to which such a relationship is held within different countries is still poorly understood. In low- or middle-income countries, higher vulnerability to climate change is associated with lower levels of wealth (Formetta and Feyen 2019), which results in higher income inequality (Palagi et al 2022). In contrast, in high-income countries, income distribution is not affected by climate change vulnerability (Cevik and Jalles 2022).
Climate change impact on inequality manifests itself through various channels. In addition to direct economic loss, climate change reduces welfare (Donadelli et al 2017) and could increase inequality within and between communities (Hsiang et al 2019). Regarding the impact on income, a study of sub-national data from 12 countries in the Americas has found an overall negative relationship between temperature and income within countries, as well as between countries (Dell et al 2009). At the global level, studies of economic inequality show that climate change exacerbates between-country inequality (Diffenbaugh and Burke 2019) as poorer countries are more vulnerable to the adverse impacts of rising temperatures. The reason is generally thought to be linked to the location of these countries in low latitudes with hot temperatures to start with (Mendelsohn et al 2006). As most of these studies have used global aggregated data on economic growth and temperature (King and Harrington 2018), only limited broad evidence is available on within-country inequality and its link to weather/climate, with a few exceptions such as a study in Vietnam (de Laubier Longuet Marx et al 2019) and another one in India (Sedova et al 2019). Both of these studies have shown that while the poor agricultural communities suffer from temperature changes, the rich are less vulnerable and more adaptive to these changes.
Highly asymmetric climate change impacts have been observed in several studies showing that more vulnerable populations in poorer developing countries of the south are affected more negatively than rich people in the developed countries of the north (Hallegatte and Rozenberg 2017, Kalkuhl and Wenz 2020, Palagi et al 2022). These findings have been challenged by more recent studies that show they hold only for mild temperature changes, while under more extreme climate projections, rich/cold countries will suffer more than hot/poor countries (Kahn et al 2019). Such inconsistency in the climate change impact literature at a global scale calls for a more detailed and local analysis of climate change damages, especially for low- or middle-income countries, where adaptation measures are absent or deemed insufficient (Mirza 2003, Mendelsohn 2008). Moreover, combining macro and micro findings is still a conceptual challenge.
This paper contributes to filling this research gap by providing a three-level analysis of the impact of weather and climate shocks on income distribution and inequality using global, regional, and household-level panel data. Our study addresses the question of climate change impact on inequality differently from other studies such as Diffenbaugh and Burke (2019) in that we provide an explicit and direct measures of inequality (e.g. the Gini coefficient and poverty indices) and show how climate change has historically affected them while the aforementioned studies rely only on country-level relationships between temperature and economic growth to measure the between-country inequality implicitly. On the other hand, recent studies that tackle climate change impact on inequality at the country level are based on the change in percentile share of each country's total net income (Paglialunga et al 2022, Castells-Quintana and McDermott 2023) while our work is the first to go beyond national accounts and use micro survey data on income distribution to estimate the within-country inequality indices at regional and household levels and show their susceptibility to climate change.
Over the past few years, several national 5 and international 6 household surveys have been continuously conducted around the world to cover the knowledge gap in the understanding of the socioeconomic dynamics of local communities and their economic behavior at the household level. Here, we use longitudinal data from five waves of NIDS conducted from 2008 to 2017 in South Africa to study the impact of temperature change on income inequality.
South Africa suffers from lasting impacts of historical racial and socioeconomic disparities which makes it one of the top countries in terms of pervasive poverty and high income inequality (Francis and Webster 2019). Empirical evidence points to several key drivers of poverty including gender, age, and income (Mdluli and Dunga 2022, Maloma and Dunga 2023). Furthermore, high unemployment rates specially among young black African population remains the greatest socioeconomic challenge facing the country's future development (Matyana and Thusi 2023). The excess supply of low-skilled labor combined with high unemployment rate in rural areas has resulted in startlingly high inequality among South Africans (Francis and Webster 2019). Meanwhile, climate change impacts are expected to be highly heterogeneous across the country with most exposed regions not always overlapping with those areas where people with higher vulnerability or lower adaptive capacity tend to live (Gbetibouo et al 2010).
South Africa has experienced a widespread increase in local and regional temperatures over the last few decades (Kruger and Shongwe 2004). Analyzing NIDS survey data in the past has revealed the non-uniform impact of climate change on the livelihood of local communities in South Africa, especially on the most vulnerable groups such as specialized crop farmers in rural areas (Turpie and Visser 2012, Tibesigwa et al 2015). Furthermore, climate shocks such as long-lasting droughts have increased food inequality among small, vulnerable farming communities (Baudoin et al 2017, Satgar and Cherry 2020). In other words, climate change may exacerbate the existing widespread inequality (Hundenborn et al 2018) and pervasive poverty (Finn and Leibbrandt 2017) in the South African society.
Figure 1 (left-panel) shows the historical trend of rising annual mean temperature and inequality (measured by the Gini coefficient) in South Africa, while the box-plot (right-panel) portrays the Gini coefficient and the mean temperature across the five waves of the survey data from South Africa used in this paper.
Figure 1. Historical trends of inequality and annual mean temperature in South Africa (sources: World Income Inequality Database (WIID), NIDS, and ERA5).
Download figure:
Standard image High-resolution image2. Conceptual framework
We follow the approach of Burke et al (2015b) (BHM henceforth), also used in Antonelli et al (2020), Shayegh et al (2020), Shayegh and Dasgupta (2022), Dasgupta and Robinson (2023a)to estimate the non-linear relationship between the annual mean temperature 7 and the annual change in an economic outcome variable (). In the case where
represents the logarithm of gross domestic product (GDP),
measures the rate of GDP growth, and we can compute the impact of climate change on a baseline growth rate for a given country. The same reasoning can be applied to (logarithm of) individual household income. As for the income distribution within a country, we consider inequality indices and in particular the Gini coefficient
. Moreover, we can extend our analysis to different poverty measures, including the poverty headcount index (P0), that is the proportion of the population that is counted as poor., the poverty gap index (P1), and the poverty severity index (P2) . Since all these indices are bounded between 0 and 1, using the measure directly as the dependent variable in the regressions would have two shortcomings: first, projections could result in values below 0 or above 1, and second, the linear model imposes a uniform marginal effect of the independent variables on the inequality measure. However, neither one point nor one percentage point increase or decrease in the Gini coefficient must necessarily be evaluated constantly across its domain; an increase by one point at an already more extreme value is both more difficult to achieve and also relevant potentially. Therefore, we use a transformation of the inequality measures, to be used as the dependent variable in our empirical analysis, which, in reversing the transformation limits its value to be between 0 and 1, and has the highest marginal increase at its midpoint while decreasing towards both extremes. In particular, we specify yit as the logit of the Gini index:

Hence, we estimate the change in the Gini coefficient using a generalized linear model with transformation and the variance of the binomial family. Because this transformation is monotonically increasing, the shape of the non-linear relationship is preserved. Moreover, we can simply compute the projected Gini based on the estimated transformed index. Notably, the goodness of fit in terms of the adjusted R2 and the relative size of the standard errors are higher with the transformed value. Once we have the impact both on GDP growth and the Gini coefficient, we can compute a welfare measure based on the Sen/Foster welfare function (Sen 1973) given by . In this welfare measure, an 'equality index' is calculated by subtracting the inequality index from one, which is multiplied by GDP per capita to yield an easy-to-interpret welfare value in monetary terms. By combining climate impacts on GDP (for instance, from BHM), we can estimate the growth rate of the second term
based on our Gini-based regressions. Consequently, the growth rate of the welfare measure Wit will be the sum of GDP per capita growth rate and of the second term based on the Gini coefficient. Therefore, we can decompose the welfare impact of warming into its impacts on GDP per capita and inequality.
2.1. Econometric framework
We use the following econometric framework to investigate the impact of temperature on various dependent variables yit , including (the logarithm of) GDP or household income per capita, the log-odds ratio transformed inequality indices, and several poverty measures.
Note that we consider different units of observation, notably country as well as district, household, and individual-level data for South Africa. We use the first difference of the dependent variable () as in BHM to estimate the following general equation:

Depending on the dataset and variable considered (e.g. GDP per capita, household income, log-odds ratio transformed inequality indices, and poverty measures), the dependent variable () captures the change in yit in country/ district/ household/ individual i in year t. We are interested in the effect of climatic shock measured by the 30-year rolling mean of temperature Tit up to the year preceding the current year t. However, we also run alternative specifications with mean annual temperature in year t to study the impact of short-term weather shocks. We utilize a quadratic specification to capture potential non-linear effects. The dependent variable may decrease 8 due to initial increases in temperature, however, beyond a threshold, incremental rises in temperature may increase inequality. If for the set of coefficients of temperature, we find
, this indicates a
-shaped relationship with an 'optimal' value of temperature computed as
. If, on the other hand,
holds, the relationship is
-shaped with a minimum of the dependent variable at
.
The term X it is the matrix of other relevant country/district-level control variables influencing income inequality. In the case of global and regional inequality analysis, we control for log of GDP per capita and its second-degree polynomial. For the district-level analysis in South Africa using the NIDS household survey, we include the log of income computed from household income and its second-degree polynomial, and the rate of employment.
We also include country/district/household/ individual level fixed-effects (αi ) capturing all time-invariant attributes affecting income inequality. These fixed effects allow us to identify the effects of annual temperature with the plausibly exogenous variation in temperature over time within countries/districts and years. The term γt represents survey wave/year fixed-effects, while εit is a random error term.
2.2. Data
We use several datasets at multiple spatial scales for the various analyses in this paper.
Global analysis: We use the World Income Inequality Database (WIID) (Solt 2016) for our country-level analysis. This dataset provides information on income inequality measures for 197 countries and four historical entities between 1940 and 2021.
Spatial analysis: We use annual gridded economic activity and population data between 1990–2015 from Kummu et al (2018). This dataset downscales both national and sub-national GDP (constant 2011 international US dollars) data based on a resolution of 5 arcmin (approximately km at the equator).
Household analysis: The data comes from five waves (2008–2017) of NIDS conducted by the Southern Africa labor and Development Research Unit (SALDRU) based at the University of Cape Town 9 . This is the first nationally representative panel study of households in South Africa and uses a stratified, two-stage cluster sample design to sample households in the nine provinces of the country. NIDS primarily examines the livelihoods of individuals and households over time and provides information on coping with shocks and includes detailed information on poverty and well-being, fertility and mortality, migration, economic activity, human capital, health, education, and social capital.
Climatic data: We use reanalyzed climate data from ERA5, the fifth generation European Centre for Medium-Range Weather Forecasts (ECMWF) atmospheric reanalysis of the global climate. The data is available at a spatial resolution of 0.25∘ × 0.25∘ and hourly temporal resolution (C3S 2017). We use population weights to aggregate the gridded climatic data to the district level in South Africa and country level.
3. Global analysis of temperature and inequality
First, we estimate the response function at the global level of the country-level (logit-transformed) Gini coefficient to warming by fitting a non-linear curve to the observed data from the year 1990 to 2016 from 157 countries 10 . The results from the regression are presented in table 1. Figure 2 shows that this transformed Gini coefficient is smooth, non-linear, and convex in temperature (for both climate and weather shocks), with a minimum at 13.1 ∘C for the 30-year rolling mean of temperature. However, these minima are estimated to be much lower at 11.5 ∘C in the case of annual mean temperature (weather shock) 11 . Interestingly, the optimal temperature in the specification using annual mean temperature is below the 13 ∘C peak reported for economic productivity by BHM (this paper uses the same specification). This result suggests that cold-country productivity increases while the inequality decreases as annual mean temperature increases until the Gini optimum of 11 ∘C is reached. From 11.5 ∘C to 13 ∘C we observe improving productivity at the cost of rising inequality. Productivity declines gradually after 13 ∘C with further warming, and with it, inequality grows at a faster pace than before. Similar to the analysis of the climate change impact on economic growth, the increase in inequality in higher temperatures can be attributed to the fact that poorer countries are more vulnerable to higher temperatures as many of them rely on agriculture as their primary source of income (Coulibaly et al 2020). Therefore, a more in-depth analysis at the regional and local scales is necessary to entangle the link between climate change and inequality. Table A1 and figure A.7 in appendix
Figure 2. Global non-linear relationship between 30-year rolling mean temperature (left-panel), mean temperature (right-panel), and Δln(Gini/(1-Gini)) (dark navy line) with 95% confidence interval (light blue area).
Download figure:
Standard image High-resolution imageTable 1. Global relationship between weather, climate shocks, and inequality.
(1) | (2) | |
---|---|---|
Δln(Gini/(1-Gini)) | ||
Log of GDP per capita | −0.030** | −0.046*** |
(0.022) | (0.001) | |
Log of GDP per capita squared | 0.001* | 0.002*** |
(0.070) | (0.002) | |
Annual mean temperature | −0.003** | |
(0.027) | ||
Annual mean temperature squared | 0.0001*** | |
(0.001) | ||
30-year rolling mean temperature | −0.025*** | |
(0.000) | ||
30-year rolling mean temperature-squared | 0.001*** | |
(0.000) | ||
Constant | 0.171*** | 0.317*** |
(0.005) | (0.000) | |
R-squared | 0.329 | 0.357 |
Adjusted R-squared | 0.286 | 0.111 |
Observations | 3108 | 3108 |
Number of countries | 156 | 156 |
p-values in parentheses | ||
*** p ![]() ![]() ![]() |
4. Regional analysis of temperature and inequality
Next, we turn to analyzing within-country inequality focusing on South Africa. South Africa is an interesting case of a developing country with large human capital and an atypical advanced economy for an African country. It also possesses a moderately warm climate that is affected by the currents of the surrounding oceans. We use gridded economic activity data from Kummu et al (2018) to investigate the impact of warming on a spatial Gini coefficient for each district using the gridded economic activity data aggregated to the sub-national level. Obviously, this method underestimates the full income distribution but allows us a first dive into within-regional inequality based on spatial data. We run the specification in equation (2) controlling for the log of GDP per capita, 30-year rolling mean temperature/annual mean temperature, and their second-degree polynomials. We find that the relationship between temperature (both measures) and spatial Gini is -shaped (figure 3 and table 2), similar to our findings from the global results reported in figure 2. For the simple change in the Gini coefficient, we report the results in appendix A.2 (see figure A.8 and table A2).
Figure 3. Non-linear relationship between 30-year rolling mean temperature (left-panel), mean temperature (right-panel), and spatial inequality in South Africa (dark navy line) with 95% confidence interval (light blue area).
Download figure:
Standard image High-resolution imageTable 2. South Africa spatial Δln(Gini/(1-Gini)) regression based on gridded data.
(1) | |
---|---|
Δln(Gini/(1-Gini)) | |
Log of GDP per capita | 0.018 |
(0.173) | |
Log of GDP per capita-squared | −0.0007*** |
(0.214) | |
Mean temperature | −0.001** |
(0.033) | |
Mean temperature-squared | 0.00 004** |
(0.045) | |
Constant | −0.114 |
(0.187) | |
R-squared | 0.126 |
Adjusted R-squared | 0.114 |
Observations | 208 |
Number of districts | 52 |
Robust p-values in parentheses | |
*** p ![]() ![]() ![]() |
Overall, the spatial data analysis for South Africa confirms the global results of a -shaped relationship for the Gini and
-shaped for GDP per capita (appendix
5. Micro-based analysis of temperature and inequality
We now proceed to use household micro-data using the NIDS dataset from South Africa to investigate the impact of warming on income inequality at the district-level. We focus on the impact of the 30-year rolling mean of temperature and annual mean temperature on various measures of inequality. Increased temperature has been shown to increase inequality at the global level (Diffenbaugh and Burke 2019). However, there is a lack of micro-based evidence on the impact of temperature on income inequality. Table 3 shows the descriptive statistics across districts.
Table 3. Descriptive statistics.
Variables | Mean | Min | Max | SD |
---|---|---|---|---|
Gini | 0.50 | 0.31 | 0.89 | 0.08 |
P0 | 0.37 | 0.03 | 0.88 | 0.20 |
P1 | 0.17 | 0.01 | 0.57 | 0.17 |
P2 | 0.10 | 0.00 | 0.41 | 0.10 |
Household income (ZAR) | 7651.0 | 1886.7 | 71 401.8 | 6065.9 |
Household expenditure (ZAR) | 3386.2 | 437.4 | 79 116.5 | 5156.8 |
Mean temperature (∘C) | 17.0 | 8.3 | 25.9 | 3.5 |
We evaluate inequality based on individual-level data aggregated to the district level. That is, we estimate inequality indices the district-level in South Africa using individual income responses over the five waves of NIDS. Individual weights from the fifth wave are used for the aggregation. Figure 4 shows the spatial distribution of Gini coefficient across South Africa over the five waves and suggests a substantial variation, both temporally and spatially.
Figure 4. Gini coefficient by district in South Africa over five waves of NIDS.
Download figure:
Standard image High-resolution imageFigure 4 shows the Gini coefficient and annual mean temperature across the 52 districts in South Africa. The plots suggest that there is significant heterogeneity in both inequality and climate across the country. Now, using the proposed transformation of the Gini coefficient as [ and using its change over time as dependent variable, we estimate the impact of both climate and weather shocks on within-country inequality in South Africa. The findings suggest that the temperature-inequality response functions for both 30-year rolling mean temperature and annual mean temperature are
-shaped (figure 5 and table 4). Income inequality in South Africa is non-linear and convex in temperature and is minimal at around 17 ∘C for annual mean temperature (weather shock). Using the 30-year rolling mean temperature to investigate the impact of climate shocks on inequality in South Africa, we estimate a much lower optimal temperature of 14.5 ∘C (figure 5; right-panel).
Figure 5. Non-linear relationship between mean temperature (left-panel), 30-year rolling mean temperature (right-panel) and Δln(Gini/(1-Gini)) (dark navy line) with 95% confidence interval (light blue area) at the district-level in South Africa.
Download figure:
Standard image High-resolution imageTable 4. Weather, climate, and inequality in South Africa.
(1) | (2) | (3) | (4) | |
---|---|---|---|---|
Full sample | Agricultural households | |||
Δln(Gini/1-Gini) | ||||
Rate of employment | 0.377** | 0.289* | 0.412** | 0.367** |
(0.044) | (0.088) | (0.021) | (0.041) | |
Log of income | 0.570** | 0.334 | 0.602** | 0.591* |
(0.039) | (0.142) | (0.029) | (0.084) | |
Log of income-squared | −0.024 | −0.010 | −0.029* | −0.026* |
(0.108) | (0.377) | (0.061) | (0.064) | |
Mean temperature | −0.070*** | −0.064*** | ||
(0.002) | (0.000) | |||
Mean temperature-squared | 0.002*** | 0.002*** | ||
(0.002) | (0.006) | |||
30-year rolling mean temperature | −0.054*** | −0.054*** | ||
(0.001) | (0.007) | |||
30-year rolling mean temperature-squared | 0.002*** | 0.002*** | ||
(0.002) | (0.000) | |||
Constant | −2.558** | −1.672 | −2.106*** | −1.008 |
(0.039) | (0.111) | (0.003) | (0.159) | |
Observations | 260 | 260 | 260 | 260 |
Number of districts | 52 | 52 | 52 | 52 |
Robust p-values in parentheses | ||||
*** p ![]() ![]() ![]() |
5.1. Potential mechanisms
One potential channel through which climate and weather shocks affects inequality in a country is through the agricultural sector. Although the agriculture, forestry, and fishing share of GDP in South Africa is 2.6% in 2022, 21% of total employment is in the agricultural sector, and 17.2% of households are involved in agricultural activities (World Bank 2023). Climate change has a relatively higher impact on agriculture through negative impacts on crop yields (Lobell et al 2011, Lobell and Asseng 2017, Roberts et al 2017), which in turn may drive up food prices, resulting in a reduction in access to food (Kangalawe 2012, Bradbear and Friel 2013, Myers et al 2017). Warming and precipitation variability also affects farm productivity and income (Taraz 2017, Aggarwal 2021). Food and nutrition security are also negatively affected by climate and weather shocks (Dasgupta and Robinson 2021, 2022). Additionally, workers in the agricultural sector are disproportionately affected by climate and weather shocks (mainly driven by heat stress), both in terms of labor supply (number of working hours) and labor productivity (Shayegh et al 2020, Dasgupta et al 2021, Shayegh and Dasgupta 2022). Given that the labor force involved in the agricultural sector have relatively lower incomes, these higher effects are likely to have distributional and inequality implications (Dasgupta et al 2021, Dasgupta and Robinson 2023b).
We identify households involved in agricultural using the agriculture module of the NIDS questionnaire. Then we run equation (2) for this set of households only. For both 30-year rolling mean temperature and annual mean temperature, the -shaped response-functions hold. Our findings suggest that agricultural households in South Africa are more vulnerable to climatic and weather shocks as the optimal temperature at which income inequality is at minimal is 16.1 ∘C for mean annual temperature (table 4; column 5) and 13.6 ∘C for the 30-year rolling mean temperature (table 4; column 6).
While our focus is on the relationship between temperature and inequality, we also examine the effects of climate and weather shocks on several poverty indices. The results on the impact on various alternative measures of inequality (see appendices -shaped while the temperature and income growth response-functions are
-shaped, suggesting that our estimations are robust across various measures and spatial scales.
6. Inequality in South Africa in the 21st century
Based on the different specifications estimated, we now proceed to simulate the effect of climate change on the Gini coefficient in the future. We use a unified shared socioeconomic pathways-representative concentration pathway (SSP-RCP) scenario framework for projecting future socioeconomic and environmental changes in South Africa throughout the century. The SSP scenarios project five different outcomes based on different approaches to sustainability, human capital development, and migration (O'Neill et al 2017).
SSP1 is the sustainability scenario with a medium level of migration and high levels of low-emission technologies development and deployment. Countries enjoy higher rates of education and human capital accumulation and inequality is reduced both across and within countries.
SSP2 is the middle of the road scenario with a medium level of migration where the world follows a business-as-usual development path. Global population grows moderately while there will be slow improvements in income inequality and environmental challenges.
SSP3 represents a world filled with regional rivalry with a low level of migration and a decline in investments in education and technological development. Slow economic development coupled with high population growth in developing countries exert large pressure on natural resources and environmental indicators.
SSP4 is the inequality scenario with medium levels of migration where economies are growing in different rates across the globe. The world will be divided between highly educated societies with access to high technologies and capital and resources and lower-income, poorly educated societies with low-tech economy.
SSP5 represents a world with a high level of migration where economic development is closely tied to high fossil-fueled consumption. Technological progress is rapid and human capital grows fast. Investments in health, education, and institutions increases as global population peaks and declines in the 21st century.
In the 'Reference' case, the Gini coefficient is computed based on projections depending upon the socioeconomic changes of each SSP scenario. For example, GDP per capita in 2100 ranges from 14 300 to 44 000 US$[2005] across the SSPs while Gini coefficient varies from 0.49 to 0.64 depending on productivity and educational developments among others (Rao et al 2018). We combine this projections with the historical data based on the Standardized World Income Inequality Database (Solt 2016) to obtain consistent trajectories of South Africa's future welfare and inequality measures across SSPs. We use these SSP Gini projections to compute the Reference growth rate of the logit of the Gini coefficient equality index .
For the climate change projections, we adopt different pathways to greenhouse gases concentration (Van Vuuren et al 2011). The four RCP scenarios (RCP2.6, RCP4.5, RCP6.0, and RCP8.5) correspond to the projected level of radiative forcing in 2100. Moreover, we show the results for the Reference projections without climate change ('Reference case'). We consider in particular the climate model trajectories RCP2.6 and RCP6.0 across all models as the ensemble mean, which indicates an increase in the annual mean temperature in South Africa from 18 ∘C in 2018 to 20.3 ∘C by 2100 in RCP6.0. Based on the empirical specifications the log-odds ratio transformed Gini coefficient in equation (2), and in analogy to the projection of GDP per capita in BHM, we can thus evaluate the change in the Gini coefficient from the reference projection based on a growth rate of the equity index as;
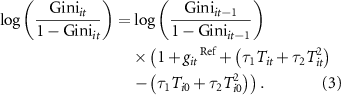
For the overall inequality level, using the best specification estimates of table 4 as , the top-left panel in figure 6 shows the Gini coefficient projections under RCP2.6 and RCP6.0 scenarios along with the Reference case without climate change. Under the RCP6.0 scenario, the Gini coefficient reaches 0.63 by 2100 in SSP2 which is higher than its values in the 'Reference' case of 0.57 indicating the role of climate change in increasing inequality. Across all SSPs, climate change (as defined by the difference between the results of RCP6.0 scenario and the 'Reference' case) increases Gini coefficient between 3 and 6 points by 2100. Note that under RCP2.6 scenario (i.e. where global mean temperature increase stays below 2 ∘C), the Gini coefficient increase compared to the 'Reference' case without climate change is much smaller (e.g. by 0.6 points under SSP2).
Figure 6. Projection of GDP per capita, equality, and welfare for South Africa decomposed.
Download figure:
Standard image High-resolution imageNext, using the Sen/Foster welfare function and thus decomposing the growth impact on GDP per capita and inequality, we use the base case SR specification from BHM to project the equality index (top-right panel in figure 6), GDP per capita (bottom-left panel in figure 6), and finally the combined effects on the welfare (bottom-right panel in figure 6). We make two main observations based on the results of these graphs. First, the GDP growth effect shows losses of around 43% of the GDP in the 'Reference' case, consistent with Burke et al (2015b) given the RCP6.0 scenario. Second, climate change increases inequality significantly and varying across SSPs. Combining these two factors, climate change is expected to reduce welfare by around 50% (49%–51% under the different SSPs) of its value under the 'Reference' case without climate change across SSP projections.
7. Conclusion
Climate change not only affects the aggregate economic productivity but also impacts income distribution and inequality. Climate change has a disproportionately higher impact on the most vulnerable population groups such as women, the elderly, children, low-income earners, and other socially marginalised groups, while poorer countries are also shown to have been relatively more affected. Climate change not only increases inequality, but increased inequality exacerbates many of the climate change induced impacts on the society through increased exposure and vulnerability. Unless climate change policies incorporate inequality in their design, they may have unintended consequences and increase the burden on disadvantaged groups. As such it is important to provide a robust evidence base on the effects of climate change on within-country inequality and poverty to identify future hotspots and to aid the design of tailored policies that integrate local contexts and local-level distributional implications. In this paper, we address the direct link between climate and weather shocks conceptually and apply it to the case of South Africa which suffers from an underlying pervasive inequality and widespread poverty.
Using data at multiple spatial scales (country, grid-cell, and household); first, we find that country-level Gini coefficient (evaluated as log-odds ratio transformed variable) is smooth, non-linear, and convex in temperature, with a minimum at about 11 ∘C in the case of annual mean temperature and 13.1 ∘C for our climate change variable measured by a 30-year rolling mean of temperature. Second, using gridded data and a nationally representative longitudinal micro-survey, we estimate that GDP/income per capita is highest at 15.6 ∘C (gridded data) and 16.8 ∘C (household survey data), respectively. Third, we investigate the impact of warming on inequality at the district level in South Africa. The results suggest that the temperature-inequality response functions are -shaped, similar to exposure-response functions for the temperature-mortality relationship (Dasgupta 2018, Dasgupta and Robinson 2023a). Income inequality in South Africa is non-linear and convex in mean temperature and is minimal at around 17 ∘C, but the minimum is much lower at 14.5 ∘C for the 30-year rolling mean temperature. While the underlying drivers of climate impacts on inequality is an area for future research, its impact on different sectors, labor supply and demand, and thus skill premiums seems a strong channel. On the other hand, fiscal policies of income redistribution are arguably not influenced directly by weather conditions. We explore the relatively higher vulnerability of the agricultural sector as a potential mechanism through which climatic and weather shocks affect inequality. A separate analysis suggests that households involved in agricultural activities have a lower temperature threshold beyond which income inequality increases.
Two main robust findings can be derived from these results; While GDP or income per capita shows a -shaped relationship with temperature, inequality and poverty relationship with temperature is
-shaped. This observation holds for all three sources of inequality from macro aggregates to micro household data. In terms of the location of the extreme, we find that the optimal temperatures are lower for inequality measures than for income levels. This indicates that substantial increases in inequality are expected at higher temperatures than growth impacts. Given that the average annual temperature in South Africa (around 18 ∘C in 2018) lies on the right side of both extreme points, all future climate change scenarios will have negative impacts on both income growth and inequality.
While the findings of this study appear to be fairly robust, and in line with other studies e.g. at the global level, we note the following limitations; (1) the main results from this paper are from South Africa, as such further research is needed in order generalize the findings, (2) while we have identified reliance on agricultural activities as one plausible mechanism through which climate and weather shocks affect inequality, identifying individual and household-specific channels is difficult due to lack of data, and (3) the projections rely on baseline inequality projections based on the SSP framework, and notably on the assumption of the estimated relationship to be stable over the 21st century
Finally, we re-emphasize the fact that South Africa is a country with deep structural socioeconomic and racial inequalities that are exacerbated by climate change. As exemplified by Cape Town's water crisis during 2015–2018, inadequate infrastructure and weak social protection policies can push the existing social inequalities to an alarming level (Millington and Scheba 2021). On the other hand, the more recent nationwide power crisis highlights the urgent need for structural reform in the power sector by moving away from old and dirty fossil fuel generation to a more decentralized system based on renewable energy, which can potentially achieve both reliability and sustainability targets (Phiri and Nyoni 2023). The co-benefits of decarbonization is more evident from our modeling exercise. When we combine the results of our empirical analysis with climate scenarios, we observe that climate change under a moderate scenario of RCP6.0 could increase the Gini coefficient by about 3–6 points compared to the 'Reference' case without climate change, while under the mitigation-driven case of RCP2.6, the increase is contained less than one point by the end of the century. This points to a less-discussed co-benefit of mitigation in terms of reducing poverty and closing the inequality gap especially in developing countries and among lower-income households. Coordinated global efforts to reduce greenhouse gas emissions can not only alleviate climate change damages and economic losses in the Global South but also contribute to better reallocation and redistribution of wealth in these countries.
Data availability statement
The data cannot be made publicly available upon publication due to legal restrictions preventing unrestricted public distribution. The data that support the findings of this study are available upon reasonable request from the authors.
Appendix A: Alternative measures of inequality
A.1. Global analysis of temperature and inequality
Figure A.7. Global non-linear relationship of temperature—inequality.
Download figure:
Standard image High-resolution imageTable A1. Global inequality regression.
(1) | |
---|---|
Δ Gini | |
Log of GDP | 0.009*** |
−0.005 | |
Log of GDP-squared | −0.0004** |
−0.019 | |
Mean temperature | −0.0006** |
(0.014) | |
Mean temperature-squared | 0.00 003*** |
(0.000) | |
Constant | −0.4555*** |
(0.001) | |
R-squared | 0.088 |
Adjusted R-squared | 0.080 |
Observations | 3108 |
Number of countries | 156 |
Robust p-values in parentheses | |
*** p ![]() ![]() ![]() |
A.2. Regional analysis of temperature and inequality
Figure A.8. Non-linear relationship of temperature—spatial inequality in South Africa.
Download figure:
Standard image High-resolution imageTable A2. South Africa spatial Gini regression based on gridded data.
(1) | |
---|---|
Δ Spatial Gini | |
Log of GDP | −0.0029*** |
(0.000) | |
Log of GDP squared | 0.00 007*** |
(0.000) | |
Mean temperature | −0.0002** |
(0.013) | |
Mean temperature-squared | 0.000 007*** |
(0.002) | |
Constant | 0.030*** |
(0.000) | |
R-squared | 0.105 |
Adjusted R-squared | 0.102 |
Observations | 208 |
Number of districts | 52 |
Robust p-values in parentheses | |
*** p ![]() ![]() ![]() |
A.3. Micro-based analysis of temperature and inequality
As an alternative specification, we estimate the 'equality index' (figure A.9) and use its growth rate (figure A.10 and table A3) as dependent variable. This specification allows to easily decompose the growth rate or the Foster/Sen welfare function into the growth rate of GPD per capita and this index.
Figure A.9. Non-linear relationship between temperature and income inequality (dark navy line) with 95% confidence interval (light blue area) at the district level in South Africa. Specification also controls for income per capita, rate of employment, and district and wave fixed-effects.
Download figure:
Standard image High-resolution imageFigure A.10. Non-linear relationship between temperature and growth in equality index (dark navy line) with 95% confidence interval (light blue area) at the district level in South Africa.
Download figure:
Standard image High-resolution imageTable A3. Temperature and Δln(1-Gini).
(1) | |
---|---|
Δln(1-Gini) | |
Rate of employment | −0.0558 |
(0.740) | |
Log of income | −0.5222* |
(0.095) | |
Log of income-squared | 0.0232 |
(0.179) | |
Mean temperature | 0.0322*** |
(0.007) | |
Mean temperature-squared | −0.0009** |
(0.017) | |
R-squared | 0.187 |
Adjusted R-squared | 0.137 |
Observations | 208 |
Number of districts | 52 |
Robust p-values in parentheses | |
*** p ![]() ![]() ![]() |
Appendix B: The impact on poverty
While the inequality indies used so far evaluate the full distribution of income, we now focus on the issue of poverty, evaluating only individuals below the poverty line. Using several poverty indices, we also find a convex relationship between temperature and poverty in South Africa, similar to results obtained for the temperature-inequality relationship (see table A4). We find that the mean temperature of minimal poverty is at 17 ∘C for the headcount index (P0), with higher values of 20.7 ∘C for the poverty gap index (P1), and 22.8 ∘C for the squared poverty gap index (P2). This result suggests that poverty is minimal at a higher temperature compared to inequality in South Africa. Our findings show that poverty declines with initial increases in temperature, however, from some point onward increase in temperature results in increased poverty at the district-level in South Africa.
Table A4. Temperature—poverty regression results.
(1) | (2) | (3) | (4) | (5) | (6) | |
---|---|---|---|---|---|---|
Δ P0 | Δ P1 | Δ P2 | Δ P0 | Δ P1 | Δ P2 | |
Rate of employment | 0.047 019 | 0.1908** | 0.0809 | 0.0635 | 0.2109* | 0.0956 |
(0.507) | (0.038) | (0.164) | (0.2727) | (0.0365) | (0.1066) | |
Log of income | 0.7138*** | 0.7977*** | 0.7524** | 0.6930*** | 0.6521*** | 0.6310*** |
(0.000) | (0.000) | (0.000) | (0.000) | (0.000) | (0.000) | |
Log of income-squared | −0.0339*** | −0.0396*** | −0.0389*** | −0.0330*** | −0.0324*** | −0.0328*** |
(0.000) | (0.000) | (0.000) | (0.000) | (0.000) | (0.000) | |
Mean temperature | −0.0226** | −0.0438*** | −0.0285*** | −0.0193** | −0.0296* | −0.0180** |
(0.020) | (0.000) | (0.000) | (0.0047) | (0.0127) | (0.0098) | |
Mean temperature-squared | 0.0007** | 0.0011** | 0.0006** | 0.0006* | 0.0007 | 0.0003 |
(0.038) | (0.010) | (0.017) | (0.0123) | (0.0982) | (0.152) | |
30-year rolling mean temperature | −0.0193** | −0.0296* | -0.0180** | |||
(0.0047) | (0.0127) | (0.0098) | ||||
30-year rolling mean temperature-square | 0.0006* | 0.0007 | 0.0003 | |||
(0.0123) | (0.0982) | (0.152) | ||||
Constant | −3.7018*** | −3.7299*** | −3.4259*** | −3.6240*** | −3.1339*** | −2.9246*** |
(0.000) | (0.000) | (0.000) | (0.000) | (0.000) | (0.000) | |
Observations | 208 | 208 | 208 | 208 | 208 | 208 |
Number of districts | 52 | 52 | 52 | 52 | 52 | 52 |
Robust p-values in parentheses | ||||||
*** p ![]() ![]() ![]() |
Appendix C: Temperature and regional income in South Africa
We use gridded economic activity data from Kummu et al (2018) based on a resolution of 5 arcmin (approximately km at the equator) to investigate the impact of warming GDP/income per capita. The results of the regional analysis of the impact of warming on GDP per capita is shown in table A5 and figure C.11. The results support previous findings on a non-linear
-shaped relationship between temperature and GDP per capita change. As South Africa has a warmer climate compared to the global average, the optimal temperature is estimated to be approximately 15.6 ∘C compared to 13 ∘C global peak reported for economic productivity by BHM. However, not all areas of South Africa experience the same impact.
Figure C.11. Non-linear relationship of temperature—GDP per capita in South Africa.
Download figure:
Standard image High-resolution imageTable A5. South Africa GDP per capita regression based on gridded data.
(1) | |
---|---|
Δ Income per capita | |
Mean temperature | 0.0069*** |
(0.003) | |
Mean temperature-squared | −0.0002*** |
(0.000) | |
Constant | −0.0487** |
(0.021) | |
R-squared | 0.619 |
Adjusted R-squared | 0.603 |
Observations | 10 452 |
Number of grid-cells | 419 |
Robust p-values in parentheses | |
*** p ![]() ![]() ![]() |
Appendix D: Micro-based analysis of temperature and income in South Africa
First, we look at income per capita replicating the cross-country analysis of BHM using equation (2) with household income per capita data (South Africa Rand—ZAR) before taxes and transfers and adjusted for working members in a given household computed from the five waves of NIDS aggregated to the district level using sampling weights. Regression results suggest a similar pattern to the BHM global pattern and the gridded results reported in section 4. The optimal temperature for household income per capita growth in South Africa is estimated at 16.8 ∘C. Second, instead of focusing on district-level average income, we exploit the panel structure of the data and focus on the impact of temperature on monthly individual income in South Africa (table A6 and figure D.12). We use the same specification in equation (2), but using data from all waves at the individual level with household fixed-effects. Similar to the cross-country GDP results, we find a -shaped relationship between monthly temperature and individual income with a maximum value of 18.8 ∘C.
Table A6. South Africa household income per capita regression based on NIDS.
(1) | |
---|---|
Δ Household income per capita | |
Mean temperature | 5.769** |
(0.036) | |
Mean temperature-squared | −0.172** |
(0.034) | |
Constant | −45.258** |
(0.037) | |
R-squared | 0.487 |
Adjusted R-squared | 0.416 |
Observations | 208 |
Number of districts | 52 |
Robust p-values in parentheses | |
*** p ![]() ![]() ![]() |
Figure D.12. Non-linear relationship of temperature and individual income in South Africa.
Download figure:
Standard image High-resolution imageFootnotes
-
*
This project has received funding from the European Union's Horizon 2020 research and innovation programme under Grant Agreement No. 821124 (NAVIGATE), the European Union's Horizon Europe research and innovation program under Grant Agreement No 101081369 (SPARCCLE), the European Union's Horizon Europe research and innovation program under Grant Agreement No. 101057554 (IDAlert). S D acknowledges support from COST Action PROCLIAS (Process-based models for Climate Impact Attribution across Sectors), funded by COST (European Cooperation in Science and Technology).
-
5
For example, National Income Dynamics Study (NIDS) in South Africa (Leibbrandt et al 2009).
-
6
-
7
We also considered precipitation but the results were never statistically significant and hence were omitted.
-
8
This relationship is expected to be reverse in the case of specifications with GDP/income per capita as the dependent variable.
-
9
-
10
The list of countries is available in the supplementary materials.
-
11
Note that since the log-odds ratio transformation is monotonically increasing, all properties about extrema directly carries over to the underlying Gini coefficient itself.