Projected changes in mean annual cycle of temperature and precipitation over the Czech Republic: Comparison of CMIP5 and CMIP6
- Department of Atmospheric Physics, Faculty of Mathematics and Physics, Charles University, Prague, Czechia
The multi-model ensembles like CMIP5 or CMIP6 provide a tool to analyze structural uncertainty of climate simulations. Currently developed regional and local climate change scenarios for the Czech Republic assess the uncertainty based on state-of-the-art Global Climate Model (GCM) and Regional Climate Model (RCM) ensembles. Present study focuses on multi-model spread of projected changes in long-term monthly means and inter-annual variability of monthly mean minimum, mean and maximum daily air temperature and monthly mean precipitation. We concentrate in more detail on the simulation of CNRM-ESM2-1, the driving GCM for the convection permitting ALADIN-Climate/CZ simulation contributing to the local scenarios in very high resolution. For this GCM, we also analyze a mini-ensemble with perturbed initial conditions to evaluate the range of internal climate variability. The results for the Czech Republic reveal minor differences in model performance in the reference period whereas quite substantial inter-generation shift in projected future change towards higher air temperature and lower summer precipitation in CMIP6 comparing to CMIP5. One of the prominent features across GCM generations is the pattern of summer precipitation decrease over central Europe. Further, projected air temperature increase is higher in summer and autumn than in winter and spring, implying increase of thermal continentality of climate. On the other hand, slight increase of winter precipitation and tendency towards decrease of summer precipitation lead to projected decrease of ombric continentality. The end of 21st century projections also imply higher probability of dry summer periods, higher precipitation amounts in the cold half of the year and extremely high temperature in summer. Regarding the CNRM-ESM2-1, it is often quite far from the multi-model median. Therefore, we strictly recommend to accompany any analysis based on the simulation of nested Aladin-CLIMATE/CZ with proper uncertainty estimate. The range of uncertainty connected to internal climate variability based on one GCM is often quite large in comparison to the range of whole CMIP6 ensemble. It implies that when constructing climate change scenarios for the Central Europe region, attention should be paid not only to structural uncertainty represented by inter-model differences and scenario uncertainty, but also to the influence of internal climate variability.
Introduction
The climate change is an essential problem of the planet Earth and associated global changes affect natural ecosystems as well as human activities. As a basis for the assessment of its effects across many Earth Science disciplines, global climate models (GCMs) are commonly used providing the tool for projections of these changes and impacts. Thus, the outputs of GCMs have become an indispensable source of information to many sectors and research fields, most prominently for studying climate system dynamics (e.g. Dai and Deng, 2022; Yang et al., 2022), evolution of past climates (e.g. Otto-Bliesner et al., 2017) and climate change projections (e.g. Belda et al., 2017; Coppola et al., 2021a; Thomas et al., 2022). However, model simulations of climate are subject to many uncertainties. Generally, the uncertainties in model outputs come from the inaccuracies of initial and boundary conditions, the parameterization of small-scale processes, and the structure of the model (Tebaldi and Knutti, 2007; Abramowitz et al., 2019). Additionally, in the case of future climate simulations, the unknown development of forcings that influence the climate system also plays a role (e.g. Meinshausen et al., 2020). Ensembles of simulations of one model with perturbed initial conditions are designed to provide an estimate of uncertainty stemming from internal climate variability, which is inevitably different in models and in the real world (e.g. Deser et al., 2012; Deser, 2020). The relative importance of uncertainty connected to internal climate variability increases with shorter temporal scale and smaller spatial scale of studied phenomena (e.g. Bassett et al., 2020; Poschlod and Ludwig, 2021). The multi-model ensembles provide a tool to analyze structural uncertainty (connected to choices of numerical schemes, spatial resolution, etc.), even though the interpretation of multi-model spread is not simple. However, the design of available ensembles in terms of model choice is not systematic and thus the commonly used model ensembles such as CMIP (Coupled Model Intercomparison Project) or CORDEX (Coordinated Regional Climate Downscaling Experiment) do not represent an independent estimate of the overall uncertainty (Abramowitz et al., 2019).
Czech Republic has joined the United Nations Framework Convention on Climate Change (UNFCCC). One of the obligations connected with the UNFCCC ratification is to observe the ongoing climate change and its impacts and to support research in the fields of climate change, its impacts, adaptation and impact mitigation. In present, this goal is approached within the project PERUN (https://www.perun-klima.cz/indexENG.html), connecting the study of local climate changes and impacts with preparation of adaptation options in several other disciplines and sectors, like hydrology, agriculture, etc. Regional and local climate change scenarios for the Czech Republic that are currently being developed within this project will try to distill all the available information, with CMIP6 GCMs (Eyring et al., 2016) being the straightforward resource. However, for regional and local applications, RCMs provide better information with added value coming from the higher resolution (Torma and Giorgi, 2020), eventually more detailed description of some processes, e.g. convection (Kendon et al., 2017). Regional climate models (RCMs) have become widely accepted as physically consistent tool for downscaling spatially coarse GCM simulations (Giorgi, 2019). RCMs inevitably need inputs from their driving GCMs and the RCM simulations are substantially influenced by the driving fields (Takayabu et al., 2016; Crhová and Holtanová, 2018; Holtanová et al., 2019; Prein et al., 2019). For the purpose of the project, EURO-CORDEX RCM simulations (e.g. Jacob et al., 2020) downscaling the CMIP5 GCMs (Taylor et al., 2012) that are available up to now are very useful. In addition, there is strong effort within the PERUN project to use convection permitting RCM ALADIN-Climate/CZ (Termonia et al., 2018; Wang et al., 2018) in very high horizontal resolution of 2.3 km and to analyze its results within uncertainty estimates derived from available ensembles of GCM and RCM simulations. Therefore, to provide a basis for further uncertainty estimate, we first analyze available GCM outputs from both the CMIP6 project and their predecessors from CMIP5 project.
In the present study, we focus on multi-model spread of projected changes in long-term monthly means and inter-annual variability of selected variables. The analysis is based on CMIP5 and CMIP6 multi-model ensembles. It is done for monthly mean minimum, mean and maximum daily air temperature and monthly mean precipitation as basic variables characterizing climate of studied area. The annual cycle of these characteristics has an important impact in various sectors of human activities as well as natural ecosystems. Some recent studies (e.g. Coppola et al., 2021a; Coppola et al., 2021b; Palmer et al., 2021) have already analyzed the CMIP5 and CMIP6 projections for Europe, but in the present study we provide a more detailed information about the expected changes focusing on Central Europe and climatic characteristics relevant for various end-users.
Besides the analysis and comparison of the whole GCM ensembles, we concentrate in more detail on the simulation of CNRM-ESM2-1 (Séférian et al., 2019) because this model (specifically the run denoted as r1i1p1f2) is used as the driving GCM in above mentioned project PERUN. For this model, we also analyze a mini-ensemble consisting of simulations with perturbed initial conditions (see Data and methods Section).
The paper is structured as follows. The data and methods used for the analysis are described in Data and methods Section. The results are described and illustrated in Results Section. Discussion Section discusses the results and Conclusion Section provides conclusions with future prospects and possible usage of our results.
Data and methods
A suite of CMIP5 and CMIP6 GCM simulations is employed here. List of all model simulations incorporated is presented in Supplementary Tables S1, S2 (in the Supplementary material). Further information and references can be found in Tebaldi et al. (2021) for CMIP6 and at PCMDI website (https://pcmdi.llnl.gov/mips/cmip5/availability.html) for CMIP5. The data were approached via Deutsches Klimarechenzentrum (DKRZ); we used monthly means of daily mean air temperature (further abbreviated as TAS), daily maximum air temperature (TX), daily minimum air temperature (TN) and daily precipitation (PR). For both CMIP5 and CMIP6, the outputs from the experiment denoted as ‘historical’ were used for the reference period 1961–1990. For the future time period of 2070–2099, we considered simulations for two alternative socio-economic and emission scenarios. For CMIP5, the representative concentration pathways (RCPs, Moss et al., 2010) RCP4.5 and RCP8.5 were used. For CMIP6, two shared socio-economic pathways (SSPs, Meinshausen et al., 2020) SSP2-4.5 and SSP5-8.5 were chosen.
The GCMs were selected based on the availability of data for selected scenarios in the time of analysis preparation. In total, there are 47 CMIP5 GCMs and 57 CMIP6 GCMs with varying number of available simulations for individual variables and time periods (Supplementary Tables S1, S2). We decided not to choose only corresponding simulations for each ensemble as we wanted to include as many model simulations as possible to gain as wide uncertainty range as possible. Where more ensemble members were available, we used only r1i1p1 simulations for CMIP5. For CMIP6 we used preferably r1i1p1f1, but where “f1” is not available, we used “f2”. The “f" index distinguishes simulations run under the same CMIP6 experiment protocol, but with different forcing. For more detailed explanation of the notation “ripf” please see CMIP protocol and associated documentation. In case of CNRM-ESM2-1 from CMIP6 ensemble we also used other available realizations (technically, ensemble members with different “r" values), varying from r1 to r10 in case of historical and SSP2-4.5, and from r1 to r5 in case of SSP5-8.5. These mini-ensembles provide a rough view of the uncertainty connected to perturbed initial conditions, e.i. representing internal climate variability, and are further denoted as “CNRM_INI”. We especially focus on the simulation of CNRM-ESM2-1 r1i1p1f2, which is the driving simulation for the Aladin-Climate/CZ as described in the Introduction Section. This simulation is denoted as CNRM_r1 in the rest of the text.
We concentrate on mean annual cycle of monthly mean values of studied variables (TAS, PR, TN, TX) and on annual cycle of standard deviation of the monthly values for both time periods 1961–1990 (further denoted as reference) and 2070–2099 (further denoted as future). Standard deviation was chosen as a basic measure of inter-annual variability of studied variables. For its calculation we used "(n-1)" in the denominator, and the data for each 30-year period were linearly detrended.
Further, we also used several observed datasets. For all incorporated variables, we used EOBS v.23.1e (Cornes et al., 2018) in two spatial resolutions (0.25° and 0.11°). For TAS and PR we also used two versions of Climatic Research Unit (CRU) datasets: TS 3.22 dataset (Harris et al., 2014) and TS 4.05 dataset (Harris et al., 2020). For PR we further used the dataset created by GPCC Global Precipitation Climatology Centre (https://www.dwd.de/EN/ourservices/gpcc/gpcc.html) version 7 in two spatial resolutions (1° and 0.5°) (Becker et al., 2013). The spread between individual observed datasets provides a benchmark for the evaluation of multi-model spread.
The GCM simulations were interpolated to a common grid corresponding to the grid used by Climatic research unit with horizontal resolution of 0.5° × 0.5°. A simple bilinear interpolation method was used for this purpose (similarly like in Belda et al., 2015). The purpose of this interpolation is to avoid the influence of different gridbox sizes which could result in different size of studied area. The observed datasets were used with their original grids.
The area used for the analysis covers the region between 11.85° and 19.15° E of longitude and 48.25° and 51.25° N of latitude (Figure 1). We concentrate on the values averaged over this region, which covers the area of the Czech Republic. The effective spatial resolution does not allow GCMs to resolve details of climate over orographically complex area of the Czech Republic (effective spatial resolution depends on numerical methods incorporated in the models but is generally several times coarser than the nominal spatial resolution), therefore we focus on areal averages over the region of interest.
The results are presented in the form of boxplots that show median, lower and upper quartiles with whiskers extending no more than 1.5*IQR (inter-quartile range) from the hinges, and outliers plotted as points.
For the comparison of model performance between corresponding pairs of CMIP5 and CMIP6 GCMs we use the root mean square error (RMSE) of monthly values plotted in Figure 2. As reference data we use the EOBS data in 0.25°. The RMSE was used for RCM evaluation over the Czech Republic by Holtanová et al. (2012), on global scale by e.g. Gleckler et al. (2008).
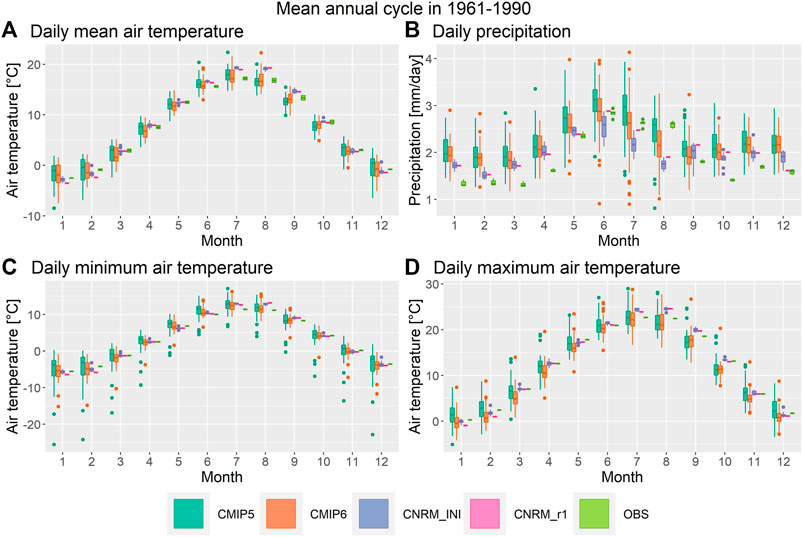
FIGURE 2. Mean annual cycle of monthly means of daily mean air temperature (A), daily precipitation amount (B), daily minimum air temperature (C) and daily maximum air temperature (D) in the period 1961–1990 averaged over the studied area (see Figure 1). Boxplots show intra-ensemble statistical distribution (median, lower and upper quartiles, whiskers representing 1.5 * IQR, and outliers as dots) of CMIP5 (green), CMIP6 (orange), perturbed initial conditions mini-ensemble of CNRM-ESM2-1 model (CNRM_INI, 10 members, blue) and observations (consisting of EOBS v. 23.1 in both 0.1° and 0.25° resolutions, for daily mean air temperature and precipitation also CRU TS 3.22 and CRU TS 4.05 datasets, for precipitation also GPCC version 7 datasets in two spatial resolutions (1° and 0.5°), green). The CNRM-ESM2-1 r1i1p1f2 simulation is plotted as a pink line.
Results
The results are described separately for the reference period of 1961–1990 (Mean annual cycle in 1961–1990 Section) and for future time period of 2070–2099 (Projected changes Section).
Mean annual cycle in 1961–1990
Figure 2 shows the results for monthly mean values of studied variables, whereas Figure 3 shows the results for annual cycle of standard deviation of the monthly values.
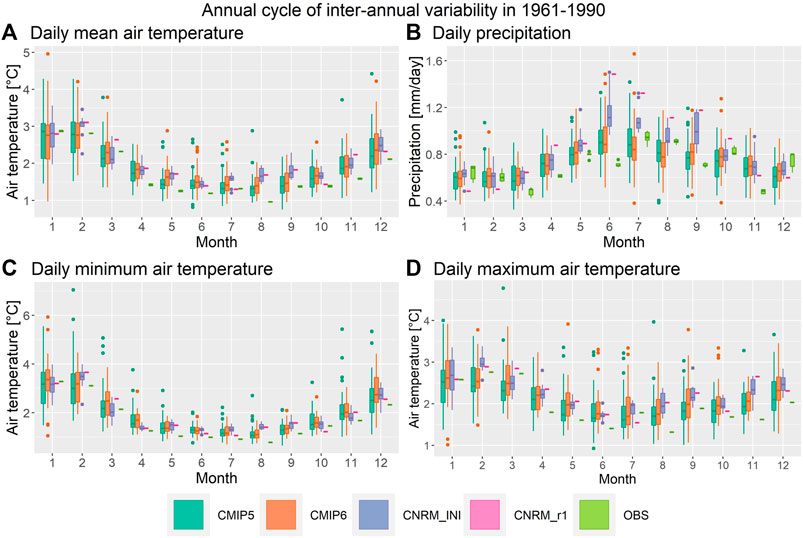
FIGURE 3. Same as Figure 2, but for standard deviation of monthly mean values.
Results for monthly means of daily mean air temperature (TAS) are displayed in Figure 2A. Generally, both CMIP6 and CMIP5 GCMs represent the mean annual cycle quite well. Except for August, September and October, the CMIP6 multi-model median gives lower temperatures than CMIP5 median, which otherwise tend to overestimate observed values. Regarding the multi-model ensemble spread (both IQR and the minimum-maximum range) it cannot be concluded that in all months it is smaller or larger in CMIP6 than CMIP5, even though there is a different number of simulations in CMIP6 (57 vs 42, see Supplementary Tables S1, S2), so it could be expected that the range of CMIP6 was larger. There are a couple of outliers in both ensembles in some months, but these are different models in different months. Therefore we cannot mark any of the GCMs to be systematically worse than the others. CNRM_INI ensemble members have mean annual amplitude of TAS higher than observed with winter temperatures lower and summer temperatures higher than observed and simulated by most of the GCMs. CNRM_r1 lies in the lower part of the multi-model distribution in January and in higher part in July and August (Figure 2A).
Both CMIP5 and CMIP6 ensembles tend to overestimate observed values of monthly mean precipitation (pr), especially in the cold half of the year (Figure 2B). Only in May, June, July and September the CMIP6 multi-model median is close to observed monthly mean precipitation. In the rest of months the differences between CMIP5 and CMIP6 are small, with CMIP6 giving slightly lower precipitation amounts (and thus being closer to observations). CNRM-INI mini-ensemble lies within the lowest quartile of CMIP6 in most months. In winter, the range of CNRM-INI mini-ensemble is comparable to the range of different observed values (from six different datasets), whereas in the rest of the year the differences between observed precipitation are much less than the range of CNRM-INI. CNRM_r1 simulation tends to be in the lower part of the CMIP6 ensemble distribution, except for May, June and July, being quite close to the observed precipitation amounts in these months (Figure 2B).
There are a few GCMs outlying strongly from both multi-model ensembles severely underestimating EOBS values of daily minimum air temperature (TN, Figure 2C, note that only EOBS dataset includes TN and TX). In CMIP5 these are all three IPSL GCMs, in CMIP6 the most outlying simulation is AWI-CM-1-1-MR, the other outliers vary between months. If we do not take these outliers into account the patterns of GCMs distributions of TN are very similar to TAS.
In case of daily maximum air temperature (TX) mean annual cycle, contrarily to TAS and TN, CMIP6 GCMs give worse results than CMIP5 in most of the months (Figure 2D). CMIP6 underestimate observed TX, whereas CMIP5 correspond well to EOBS except for August–October, where it underestimates EOBS similarly to CMIP6. A few outliers from both muti-model ensembles overestimate TX. In most of the months, these outliers correspond to the simulations that underestimate TN (see above), i.e., “IPSL” models from CMIP5 and AWI-CM-1-1-MR from CMIP6. However, it is not possible to conclude that these models would be the worst in general, because according to simulated annual cycle of TAS and PR they give better results.
Both CMIP5 and CMIP6 ensembles capture the shape of annual cycle of standard deviation of TAS quite well with respect to the observed values, with slight overestimation especially in spring and autumn (Figure 3A). Again, the outlying simulations differ between months. CNRM_INI tend to overestimate observed standard deviation of TAS, lying mostly in higher part of the CMIP6 distribution, except for January, March, April and June.
In case of standard deviation of PR one of the prominent features in Figure 3B is that the differences between observed datasets are comparable to multi-model spread in winter (DJF), the simulated annual amplitude is lower than observed and the shape of the annual cycle is not captured very well by neither of the multi-model ensembles. CNRM_INI tend to overestimate observed values with CNRM_r1 lying mostly on the edges of the multi-model spread (Figure 3B).
Mean annual cycle of standard deviation of TN is relatively well reproduced with CMIP6 having tendency to rather higher values and more overestimation than CMIP5 (Figure 3C). Similarly to mean annual cycle of TN (Figure 2C), there are a few distinct outliers, with the “IPSL” CMIP5 GCMs being within them in most of the months. CNRM_r1 overestimates observed standard deviation of TN throughout the year, sometimes being close to the CMIP6 multi-model median, sometimes in the upper part of the distribution (Figure 3C).
CMIP6 GCMs tend to simulate higher standard deviation of TX with multi-model median being thus further from observations than CMIP5 GCMs (Figure 3D). This feature is quite similar to mean values of TX in the sense that CMIP6 give slightly worse results than CMIP5 (Figure 2D). CNRM_r1 also tends to overestimate observed standard deviation of TX, except for July (Figure 3D).
For standard deviation of all studied variables, the spread of CNRM_INI is comparable to multi-model spread of CMIP6, at least in some months (Figure 3). Similar conclusion can be inferred for mean annual cycle of PR (Figure 2B). For monthly mean TAS, TN and TX the contrary is true (Figures 2A,C,D). Thus, the magnitude of uncertainty resulting from internal climate variability is large in case of variability of studied variables and also for mean values of precipitation.
For TAS and PR, observational uncertainty can be evaluated. Regarding monthly mean air temperature, there are visible differences between CRU and EOBS up to 0.9°C. However, the values of standard deviation of TAS differ much less, the differences in individual months are up to 0.2°C. For mean precipitation the uncertainty connected to observations is up to 0.1 mm/day, i.e., 3 mm per month. Generally, in comparison to multi-model spread the observational uncertainty of TAS and PR is small, except for standard deviation of precipitation in winter, where the differences between observed datasets are comparable to multi-model spread. For TN and TX only EOBS in 0.25° and 0.11° datasets are available and they give practically identical values, therefore the observational uncertainty cannot be evaluated in the present study.
To investigate more the difference in model performance between CMIP5 and CMIP6 ensembles, we compare a simple performance metric RMSE of the mean annual cycle of four studied variables for ten pairs of GCMs from both ensembles for which the CMIP5 GCM can be considered as predecessor of corresponding CMIP6 GCM (Table 1). For most of these ten pairs, the CMIP6 RMSE is lower than RMSE of corresponding CMIP5 GCM. In some cases the progress is not very high, there are also pairs with higher CMIP6 RMSE (worse performance than CMIP5). Generally, for annual cycle of PR the differences in RMSE are lower. The better CMIP6 performance is most visible for TN and TX (Table 1).
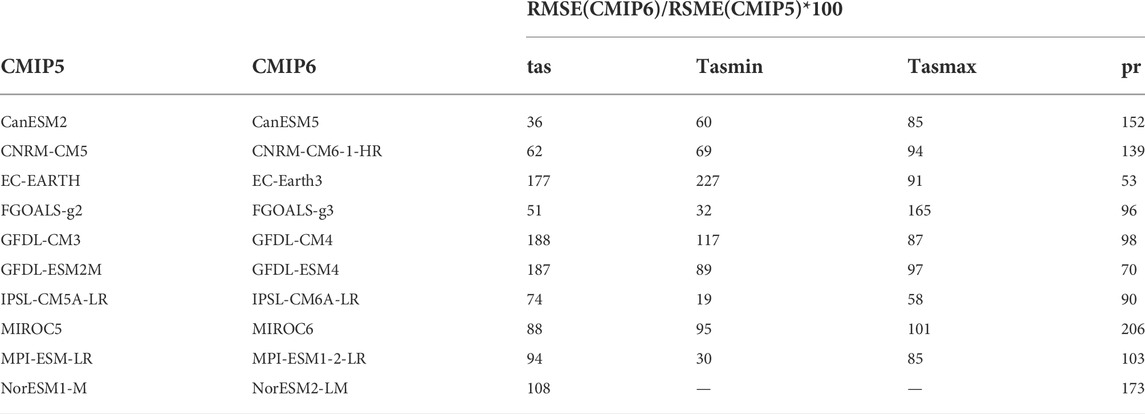
TABLE 1. Comprasion of root mean square error (RMSE) of the mean annual cycle of studied variables between selected CMIP6 and CMIP5 GCM pairs. The differences are shown relatively, values lower than 100 implying better performance of CMIP6 GCM than its CMIP5 counterpart.
Projected changes
Figure 4 shows the results for projected changes in monthly mean values of studied variables, whereas Figure 5 shows results for standard deviation of the monthly values. Changes in annual mean of monthly mean values of studied variables are also shown in Figure 4.
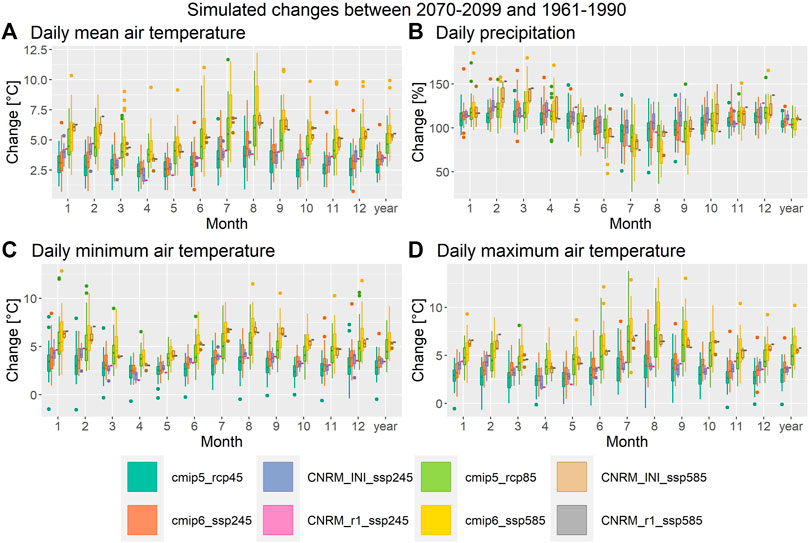
FIGURE 4. Projected changes of monthly means of daily mean air temperature (A), daily precipitation amount (B), daily minimum air temperature (C) and daily maximum air temperature (D) for the period of 2070–2099 in comparison to 1961–1990 averaged over the area of the Czech Republic, “year” denotes annual mean change. Boxplots show intra-ensemble statistical distribution (median, lower and upper quartiles, whiskers representing 1.5 * IQR, and outliers as dots) of CMIP5 under RCP4.5 (dark green), CMIP6 under SSP2-4.5 (orange), perturbed initial conditions mini-ensemble of CNRM-ESM2-1 GCM under SSP2-4.5 (10 members, blue), CMIP5 under RCP8.5 (light green), CMIP6 under SSP5-8.5 (yellow), perturbed initial conditions mini-ensemble of CNRM-ESM2-1 GCM under SSP5-8.5 (5 members, brown). The CNRM-ESM2-1 r1i1p1f2 simulation is plotted as a pink line for SSP2-4.5 and as grey line for SSP5-8.5.
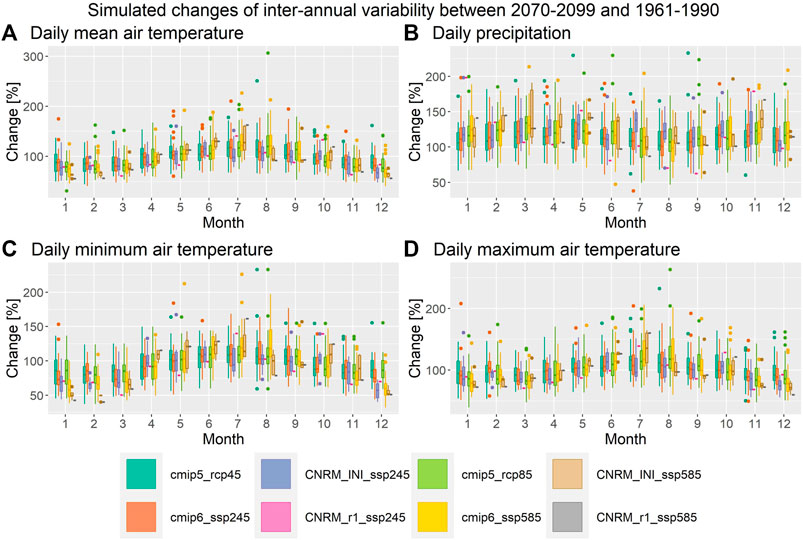
FIGURE 5. Same as Figure 4, but for relative changes in standard deviation of studied variables.
Projected changes in TAS, TN and TX are positive indicating expected increase of air temperature in studied area in all months (Figures 4A,C,D). The increase is naturally larger for stronger forcing scenarios (RCP8.5, SSP5-8.5) in all months, and in most cases the changes in summer and autumn months are higher than in winter and spring. CMIP6 project generally higher air temperature changes than CMIP5. The difference between CMIP5 and CMIP6 is higher in summer and autumn, especially for TAS. Regarding changes of TAS, TN and TX simulated by CNRM_r1, the annual mean values are very close to the CMIP6 median (Figure 4). In winter, this simulation tends to be in the upper part of CMIP6 distribution and in summer in the lower part.
Projected mean annual change of PR shows slight increase of precipitation amount with 75% of GCMs giving positive annual mean change and multi-model medians projecting change of approximately 5% for both CMIP5 and CMIP6 under both forcing scenarios (Figure 4B). CNRM_INI mini-ensemble gives higher changes around 10% (Figure 4B). However, the change is not distributed uniformly throughout the year. From November to May all GCM simulations agree on precipitation increase. During summer season, the results differ for both GCM ensembles and forcing scenarios. For CMIP5 under RCP4.5 the multi-model median change is close to zero (corresponding to value of 100% in Figure 4B, with the term “zero change” meaning no increase nor decrease of PR) and the spread between lower and upper quartiles includes zero change from June to October. Similar conclusion applies for CMIP5 under RCP8.5, with lower (more negative) multi-model median change and larger spread in July and August (Figure 4B). Under SSP2-4.5 CMIP6 projected median precipitation change is near zero in June, and from July to September slightly negative with multi-model inter-quartile spread including zero change. Under SSP5-8.5 CMIP6 GCMs project precipitation decrease from June to September with maximum median decrease of 25% in August. In October CMIP6 projected precipitation changes do not practically differ between scenarios with median changes indicating increase of approximately 5% but MME spread between quartiles involving zero change (Figure 4B). CNRM_INI gives more positive precipitation changes than most of other GCMs in all months, with practically zero median change in July and rather positive changes in other months. CNRM_r1 projects precipitation decrease of 10–25% from June to September, and precipitation increase of similar magnitude range in other months, with only minor differences between forcing scenarios.
Regarding the projected changes in inter-annual variability of TAS, TN, TX and PR, which were evaluated based on relative changes in standard deviation (Figure 5), the differences between CMIP5 and CMIP6 and individual emission scenarios are smaller than in case of changes in mean values of studied variables (compare to Figure 4). Further, the spread of CNRM_INI mini-ensemble is comparable to the spread of the whole CMIP5 and CMIP6 ensembles. This implies that the internal variability seems to play an important role in changes of inter-annual variability of studied variables. Standard deviation of TAS, TN and TX is expected to increase in summer and decrease in winter. Standard deviation of PR is projected to increase, but the multi-model spread includes zero in some months. CNRM_r1 tend to be rather far from the multi-model median of CMIP6, especially for changes in standard deviation of PR.
Furthermore, regarding the changes in standard deviation of studied variables, there are some severely outlying points visible in Figure 5, with changes up to 150–200% in several extreme cases. The outlying models vary. It is not possible to depict several particular models. However, we have to note that the CNRM_r1 is also in some cases among these outlying simulations, especially for standard deviation of PR, as already noted above.
Similarly like in Mean annual cycle in 1961–1990 Section, to investigate more the difference between air temperature changes projected by CMIP5 and CMIP6 ensembles, we compare changes in daily mean air temperature for ten pairs of GCMs from both ensembles for which the CMIP5 GCM can be considered as predecessor of corresponding CMIP6 GCM (Table 2). For the sake of easier interpretation, we only show the differences for summer and annual means of projected TAS changes. We choose summer season because the results in Figure 4 show that for this season the differences in TAS changes between the two multi-model ensembles are largest. For six out of these ten pairs, the CMIP6 signal is higher for both forcing scenarios. For “MIROC”, “FGOALS” and “GFDL-CM” pairs the difference between generations is zero or CMIP5 version gives higher TAS change than CMIP6. Also for “NorESM” pair under the lower radiative forcing the CMIP5 projected annual mean TAS change is slightly higher than CMIP6 (Table 2). Therefore, we cannot conclude that all CMIP6 GCMs give higher TAS changes than their CMIP5 predecessors do.
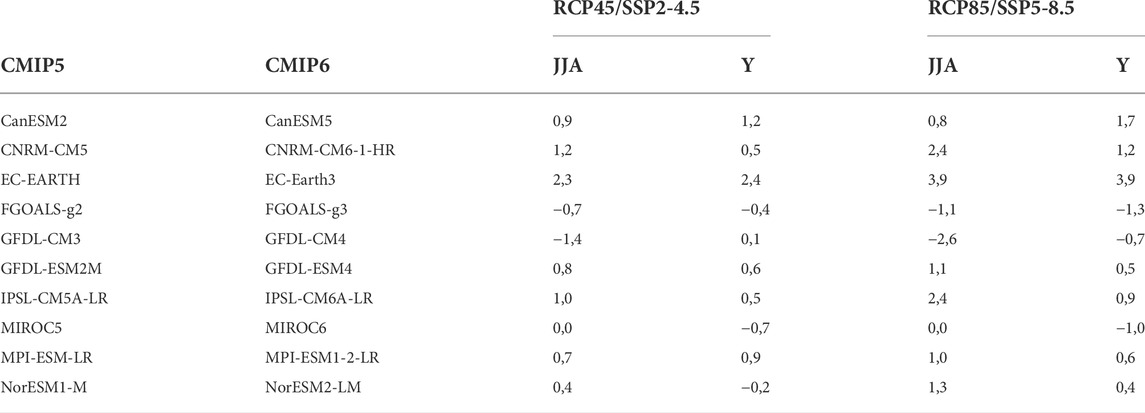
TABLE 2. Differences in TAS projected changes (°C) between selected CMIP6 a CMIP5 GCM pairs for two forcing scenarios. Positive values imply higher projected changes in CMIP6 than in CMIP5. JJA denotes mean over June-July-August season, Y denotes annual mean.
Discussion
Most of the results are presented in the form of boxplots for individual ensembles. When comparing the boxplots we have to keep in mind that each ensemble has different number of members. So for example in some cases higher number of simulations included can cause larger spread. On the other hand, we can conclude that higher number of models in CMIP6 comparing to CMIP5 does not automatically lead to larger spread, which implies higher convergence in CMIP6.
Differences in model performance and changes projected by CMIP6 simulations in comparison to previous CMIP5 GCMs are hard to attribute to one particular feature or difference between the multi-model ensembles, because the ensembles differ in various aspects. These include different number of contributing models, differences in model complexity, e.g., inclusion of wider scale of bio-geo-chemical processes in some CMIP6 earth-system models, differences in forcing scenarios etc. Regarding the forcing scenarios, according to Gidden et al. (2019) there are slight differences in emission and concentration pathways between corresponding SSP-RCP pairs in the course of the 21st century. As discussed in Tebaldi et al. (2021) there are only minor differences in the radiative forcing according to more traditional definition established by IPCC AR5 (Myhre et al., 2013). However, when considering effective radiative forcing (Smith et al., 2020), some differences arise, due to above mentioned differences in emission pathways (for detailed discussion see Tebaldi et al., 2021, Section 3.1.3).
Another important issue potentially contributing to revealed differences in projected climate changes is higher effective climate sensitivity in some of the CMIP6 GCMs (e.g., Zelinka et al., 2020) leading to higher projected temperature changes especially in summer (e.g., Palmer et al., 2021). Moreover, a number of recent studies have shown that there is a certain relationship between model performance in recent decades and the magnitude of projected future changes (e.g., Tokarska et al., 2020; Hegerl et al., 2021). When some kind of constraint is applied based on the historical simulations then the constrained CMIP6 projected changes get more in line with CMIP5 constrained projections (e.g., Tokarska et al., 2020; Tebaldi et al., 2021). However, most of relevant studies concentrate on global mean surface air temperature (e.g., Brunner et al., 2020; Tokarska et al., 2020), so the effect of such constraints over Central Europe needs to be assessed. Analysis of possible constraints is out of scope of the present study, the authors are working on a follow-up study focusing on this issue. Preliminary results for the reference period analyzed here do not show any difference in simulated air temperature trends between CMIP5 and CMIP6. Nevertheless, we can make a note, that both GCM generations tend to overestimate observed air temperature trends, with observed trends of monthly mean air temperature being mostly statistically insignificant and simulated trends statistically significant in approximately half of studied cases (not shown).
Regarding model performance, some previous studies compared the GCMs contributing to both CMIP5 and CMIP6 in order to evaluate explicitly the progress between the generations. For example, Cannon (2020) evaluated 15 such pairs of GCMs according to their skill in simulating observed patterns of atmospheric circulation over six selected continental regions based on daily values of sea-level pressure. They concluded that the simulated atmospheric circulation is substantially improved in the new generation of models. Similarly, Fernandez-Granja et al. (2021) also report a progress to better results in CMIP6 when evaluating atmospheric circulation based on Lamb classification over Europe for nine pairs of GCMs. Evaluation of model performance is not the main goal of this study, as we evaluate mainly multi-model characteristics rather than individual model performance. Anyway, our results for the reference period are quite inconclusive; there are not large differences in the resemblance with observed values between CMIP5 and CMIP6 (Figures 2, 3). On the other, the simple comparison of RMSE of mean annual cycle of studied variables for selected CMIP5-CMIP6 pairs show general tendency towards better performance in the new GCM generation.
One of the prominent features visible in our results across GCM generations is the pattern of summer precipitation decrease over central Europe. It has already been described based on both GCM and RCM projections (e.g., Coppola et al., 2021a) and has been reported to get even more prominent in CMIP6 (Palmer et al., 2021). Another noticeable feature is that projected air temperature increase is higher in summer and autumn than in winter and spring (Figure 4A). This implies a possible change in the annual air temperature amplitude and hence thermal continentality of climate. Continentality of climate generally characterizes the influence of the distance of ocean on the climate of a place (Driscoll and Yee Fong, 1992). Previous studies based on Köppen-Trewartha climatic classification showed projected transition from continental temperate climate to oceanic temperate climate over Central Europe (Feng et al., 2012; Belda et al., 2017). This is seemingly in contrast to our results. However, Köppen-Trewartha climatic classification distinguishes the continental and oceanic temperate climates based solely on a numerical threshold for air temperature of the coldest month (Belda et al., 2014). However, thermal continentality indices are generally based on annual air temperature amplitude (e.g., Gorczynski, 1922). Hence, as our results for both CMIP5 and CMIP6 show increasing annual temperature amplitude, it implies increase of continentality of climate over Central Europe. On the other hand, a different view on continentality can be based on the annual course of precipitation (e.g., Brázdil et al., 2009). More uniform annual course implies ombric oceanity, whereas increasing differences between seasonal precipitation amounts imply increasing ombric continentality. Our results show slight increase of winter precipitation and tendency towards decrease of summer precipitation (Figure 4B). Combining these projections with observed annual course of precipitation (Figure 2B), we can conclude that our results indicate projected decrease of ombric continentality over Central Europe, contrasting with expected increase of thermal continentality of climate.
Regarding projected changes in inter-annual variability of studied parameters, our results indicate increase of variability of precipitation, except for summer months, where the results are inconclusive with multi-model ranges including zero changes (Figure 5B). Together with projected decrease of summer precipitation (Figure 4B), this might lead to higher probability of dry summer periods. Increased inter-annual variability together with increased mean precipitation in winter, spring and autumn leads to higher probability of extreme precipitation that might point toward higher probability of floods. Inter-annual variability of studied temperature characteristics in summer is expected to increase (Figures 5A,C,D) which together with projected increase of monthly mean values (Figures 4A,C,D) leads to even higher probability of extremely high temperatures with possible more frequent heat waves in summer. Higher probability of high temperature extremes in future has also been concluded in previous studies, most recently e.g., in Coppola et al. (2021b).
Conclusion
We have evaluated differences between CMIP5 and CMIP6 GCM multi-model ensembles with regard to mean annual cycle of four basic climatic variables and their inter-annual variability in the 1961–1990 reference period and projected changes in 2070–2099 under two forcing scenarios. The reference period of 1961–1990 was selected to represent relatively stable recent climate over the Czech Republic. More recent reference period would be more influenced by contemporary anthropogenic climate changes (e.g., Brázdil et al., 2022). A special attention has been paid to the simulation of CNRM-ESM2-1, one of the CMIP6 GCMs, and its perturbed-initial conditions mini-ensemble. The results for the area centered on the Czech Republic reveal minor differences in model performance in the reference period whereas quite substantial inter-generation shift in projected future change towards higher air temperature and lower summer precipitation in the new GCM generation. Based on our simple comparison of selected predecessor-successor pairs it cannot be concluded that all CMIP6 GCMs project systematically higher air temperature changes (Table 2). However, the multi-model characteristics imply generally higher changes for CMIP6 GCMs, especially in summer.
Regarding the consistency of GCM simulations with observed values in the reference period, we can conclude that based on the multi-model characteristics the GCMs are capable of capturing the main features of annual cycles of studied climatic elements. Even though the model performance does not automatically imply reliability of future climate change projections (e.g., Abramowitz et al., 2019), it is a necessary condition for the process of climate change scenario creation.
Regarding the CNRM-ESM2-1, the driving GCM simulation of Aladin-CLIMATE/CZ, which is the core simulation for the PERUN climate change scenarios, its results are often quite far from the multi-model median. Therefore, we strictly recommend accompanying any analysis based on the simulation of Aladin-CLIMATE/CZ with proper uncertainty estimate using available GCMs and RCMs. On the other hand, the nested RCM does not automatically inherit the manner of behavior from the driving GCM, even the climate change signal is often substantially modified (e.g., Sørland et al., 2018; Crhová and Holtanová, 2019). We concentrated here on the CNRM-ESM2-1 simulation for the area of the Czech Republic, which lies in the center of the Aladin-CLIMATE/CZ integration domain. However, for the resulting RCM simulation, the input from GCM in the form of boundary conditions is very important (e.g., Christensen and Kjellström, 2020; Ahrens and Leps, 2021). Therefore, in an upcoming study, we will concentrate on evaluation of CNRM-ESM2-1 with regard to the boundary conditions provided to Aladin-CLIMATE/CZ, i.e., the upper-air parameters over the boundaries of the integration domain.
The range of CNRM_INI mini-ensemble represents a rough estimate of uncertainty related with internal climate variability. It is relatively larger in case of inter-annual variability of studied air temperature variables than for the mean values, in both the reference period and with regard to projected changes. For precipitation, the role of internal variability is more pronounced than for air temperature, in both reference and future time periods. The CNRM_INI mini-ensemble includes only ten or five simulations for only one model, and therefore provided uncertainty estimate must be considered as a lower bound. With regard to this fact, we can conclude that the CNRM_INI range is often quite large in comparison to the range of whole CMIP6 ensemble. It implies that when constructing climate change scenarios for Central Europe, attention must be paid not only to structural uncertainty represented by inter-model differences and scenario uncertainty, but also to the influence of internal climate variability.
Our results and above discussion lead us to the conclusion that it is necessary to explore possible constraints on simulated changes before the CMIP6 GCM outputs are used for any kind of further analysis, including uncertainty estimates for impact and adaptation studies on regional and local scales. Therefore, within the project PERUN, a further analysis of possible links between simulated changes, model performance and model climate sensitivity will be performed to tackle this issue appropriately.
Data availability statement
Publicly available datasets were analyzed in this study. This data can be found here: https://esgf-node.llnl.gov/projects/esgf-llnl/.
Author contributions
EH designed the study and wrote the paper. MB participated in data processing and calculation of the results. MB and TH helped write the paper and interpret the results. All authors contributed to the article and approved the submitted version.
Funding
The research was funded by the Technology Agency of the Czech Republic (Grant No. SS02030040, PERUN and Grant No. SS04030013 SEEPIA).
Acknowledgments
This work used resources of the Deutsches Klimarechenzentrum (DKRZ) granted by its Scientific Steering Committee (WLA) under project MCS LOVE CCS in framework of IS-ENES3 Analysis Platform activity. We acknowledge the World Climate Research Programme’s Working Group on Coupled Modelling, which is responsible for CMIP, and we thank the climate modeling groups for producing and making available their model outputs. For CMIP the US Department of Energy’s Program for Climate Model Diagnosis and Intercomparison provides coordinating support and led development of software infrastructure in partnership with the Global Organization for Earth System Science Portals. We acknowledge the E-OBS dataset from the EU-FP6 project UERRA (https://www.uerra.eu) and the Copernicus Climate Change Service, and the data providers in the ECA&D project (https://www.ecad.eu). We acknowledge the Climatic Research Unit (CRU) for creation of datasets TS 3.22 dataset (Harris et al., 2014) and TS 4.05 dataset (Harris et al., 2020). We also acknowledge the GPCC Global Precipitation Climatology Centre (https://www.dwd.de/EN/ourservices/gpcc/gpcc.html) version 7 (Becker et al., 2013).
Conflict of interest
The authors declare that the research was conducted in the absence of any commercial or financial relationships that could be construed as a potential conflict of interest.
Publisher’s note
All claims expressed in this article are solely those of the authors and do not necessarily represent those of their affiliated organizations, or those of the publisher, the editors and the reviewers. Any product that may be evaluated in this article, or claim that may be made by its manufacturer, is not guaranteed or endorsed by the publisher.
Supplementary material
The Supplementary Material for this article can be found online at: https://www.frontiersin.org/articles/10.3389/feart.2022.1018661/full#supplementary-material
References
Abramowitz, G., Herger, N., Gutmann, E., Hammerling, D., Knutti, R., Leduc, M., et al. (2019). ESD reviews: Model dependence in multi-model climate ensembles: Weighting, sub-selection and out-of-sample testing. Earth Syst. Dyn. 10 (1), 91–105. doi:10.5194/esd-10-91-2019
Ahrens, B., and Leps, N. (2021). Sensitivity of convection permitting simulations to lateral boundary conditions in idealized experiments. J. Adv. Model. Earth Syst. 13, e2021MS002519. doi:10.1029/2021MS002519
Bassett, R., Young, P. J., Blair, G. S., Samreen, F., and Simm, W. (2020). A large ensemble approach to quantifying internal model variability within the WRF numerical model. J. Geophys. Res. Atmos. 125 (7), e2019JD031286. doi:10.1029/2019JD031286
Becker, A., Finger, P., Meyer-Christoffer, A., Rudolf, B., Schamm, K., Schneider, U., et al. (2013). A description of the global land-surface precipitation data products of the Global Precipitation Climatology Centre with sample applications including centennial (trend) analysis from 1901–present. Earth Syst. Sci. Data 5 (1), 71–99. doi:10.5194/essd-5-71-2013
Belda, M., Holtanová, E., Halenka, T., and Kalvová, J. (2014). Climate classification revisited: From Köppen to Trewartha. Clim. Res. 59, 1–13. doi:10.3354/cr01204
Belda, M., Holtanová, E., Halenka, T., Kalvová, J., and Hlávka, Z. (2015). Evaluation of CMIP5 present climate simulations using the Köppen-Trewartha climate classification. Clim. Res. 64, 201–212. doi:10.3354/cr01316
Belda, M., Holtanová, E., Kalvová, J., and Halenka, T. (2017). Global warming-induced changes in climate zones based on CMIP5 projections. Clim. Res. 71 (1), 17–31. doi:10.3354/cr01418
Brázdil, R., Trnka, M., Dobrovolný, P., Chromá, K., Hlavinka, P., and Žalud, Z. (2009). Variability of droughts in the Czech republic, 1881–2006. Theor. Appl. Climatol. 97 (3), 297–315. doi:10.1007/s00704-008-0065-x
Brázdil, R., Zahradníček, P., Dobrovolný, P., Řehoř, J., Trnka, M., Lhotka, O., et al. (2022). Circulation and climate variability in the Czech republic between 1961 and 2020: A comparison of changes for two “normal” periods. Atmosphere 13 (1), 137. doi:10.3390/atmos13010137
Brunner, L., Pendergrass, A. G., Lehner, F., Merrifield, A. L., Lorenz, R., and Knutti, R. (2020). Reduced global warming from CMIP6 projections when weighting models by performance and independence. Earth Syst. Dyn. 11 (4), 995–1012. doi:10.5194/esd-11-995-2020
Cannon, A. J. (2020). Reductions in daily continental-scale atmospheric circulation biases between generations of global climate models: CMIP5 to CMIP6. Environ. Res. Lett. 15 (6), 064006. doi:10.1088/1748-9326/ab7e4f
Christensen, O. B., and Kjellström, E. (2020). Partitioning uncertainty components of mean climate and climate change in a large ensemble of European regional climate model projections. Clim. Dyn. 54 (9), 4293–4308. doi:10.1007/s00382-020-05229-y
Coppola, E., Nogherotto, R., Ciarlo, J. M., Giorgi, F., van Meijgaard, E., Kadygrov, N., et al. (2021a). Assessment of the European climate projections as simulated by the large EURO‐CORDEX regional and global climate model ensemble. JGR. Atmos. 126, e2019JD032356. doi:10.1029/2019JD032356
Coppola, E., Raffaele, F., Giorgi, F., Giuliani, G., Xuejie, G., Ciarlo, J. M., et al. (2021b). Climate hazard indices projections based on CORDEX-CORE, CMIP5 and CMIP6 ensemble. Clim. Dyn. 57 (5), 1293–1383. doi:10.1007/s00382-021-05640-z
Cornes, R., van der Schrier, G., van den Besselaar, E. J. M., and Jones, P. D. (2018). An ensemble version of the E-OBS temperature and precipitation datasets. J. Geophys. Res. Atmos. 123, 9391–9409. doi:10.1029/2017JD028200
Crhová, L., and Holtanová, E. (2018). Simulated relationship between air temperature and precipitation over Europe: Sensitivity to the choice of RCM and GCM. Int. J. Climatol. 38 (3), 1595–1604. doi:10.1002/joc.5256
Crhová, L., and Holtanová, E. (2019). Temperature and precipitation variability in regional climate models and driving global climate models: Total variance and its temporal‐scale components. Int. J. Climatol. 39 (3), 1276–1286. doi:10.1002/joc.5876
Dai, A., and Deng, J. (2022). Recent Eurasian winter cooling partly caused by internal multidecadal variability amplified by Arctic sea ice-air interactions. Clim. Dyn. 58, 3261–3277. doi:10.1007/s00382-021-06095-y
Deser, C. (2020). Certain uncertainty: The role of internal climate variability in projections of regional climate change and risk management. Earth's. Future 8, e2020EF001854. doi:10.1029/2020EF001854
Deser, C., Phillips, A., Bourdette, V., and Teng, H. (2012). Uncertainty in climate change projections: The role of internal variability. Clim. Dyn. 38, 527–546. doi:10.1007/s00382-010-0977-x
Driscoll, D. M., and Fong, J. M. Y. (1992). Continentality: A basic climatic parameter re‐examined. Int. J. Climatol. 12 (2), 185–192. doi:10.1002/joc.3370120207
Eyring, V., Bony, S., Meehl, G. A., Senior, C. A., Stevens, B., Stouffer, R. J., et al. (2016). Overview of the coupled model intercomparison project phase 6 (CMIP6) experimental design and organization. Geosci. Model. Dev. 9 (5), 1937–1958. doi:10.5194/gmd-9-1937-2016
Feng, S., Ho, C. H., Hu, Q., Oglesby, R. J., Jeong, S. J., and Kim, B. M. (2012). Evaluating observed and projected future climate changes for the Arctic using the Köppen-Trewartha climate classification. Clim. Dyn. 38, 1359–1373. doi:10.1007/s00382-011-1020-6
Fernandez-Granja, J. A., Casanueva, A., Bedia, J., and Fernandez, J. (2021). Improved atmospheric circulation over Europe by the new generation of CMIP6 Earth system models. Clim. Dyn. 56 (11), 3527–3540. doi:10.1007/s00382-021-05652-9
Gidden, M. J., Riahi, K., Smith, S. J., Fujimori, S., Luderer, G., Kriegler, E., et al. (2019). Global emissions pathways under different socioeconomic scenarios for use in CMIP6: A dataset of harmonized emissions trajectories through the end of the century. Geosci. Model. Dev. 12 (4), 1443–1475. doi:10.5194/gmd-12-1443-2019
Giorgi, F. (2019). Thirty years of regional climate modeling: Where are we and where are we going next? J. Geophys. Res. Atmos. 124, 2018JD030094–5723. doi:10.1029/2018JD030094
Gleckler, P. J., Taylor, K. E., and Doutriaux, C. (2008). Performance metrics for climate models. J. Geophys. Res. 113 (D6), D06104. doi:10.1029/2007JD008972
Gorczynski, L. (1922). The calculation of the degree of continentality. Mon. Weather Rev. 50, 369–370.
Harris, I., Jones, P. D., Osborn, T. J., and Lister, D. H. (2014). Updated high-resolution grids of monthly climatic observations—The CRU TS3.10 dataset. Int. J. Climatol. 34, 623–642. doi:10.1002/joc.3711
Harris, I., Osborn, T. J., Jones, P., and Lister, D. (2020). Version 4 of the CRU TS monthly high-resolution gridded multivariate climate dataset. Sci. Data 7, 109. doi:10.1038/s41597-020-0453-3
Hegerl, G. C., Ballinger, A. P., Booth, B. B., Borchert, L. F., Brunner, L., Donat, M. G., et al. (2021). Toward consistent observational constraints in climate predictions and projections. Front. Clim. 3, 678109. doi:10.3389/fclim.2021.678109
Holtanová, E., Mendlik, T., Koláček, J., Horová, I., and Mikšovský, J. (2019). Similarities within a multi-model ensemble: Functional data analysis framework. Geosci. Model. Dev. 12, 735–747. doi:10.5194/gmd-12-735-2019
Holtanová, E., Mikšovský, J., Kalvová, J., Pišoft, P., and Motl, M. (2012). Performance of ENSEMBLES regional climate models over Central Europe using various metrics. Theor. Appl. Climatol. 108, 463–470. doi:10.1007/s00704-011-0542-5
Jacob, D., Teichmann, C., Sobolowski, S., Katragkou, E., Anders, I., Belda, M., et al. (2020). Regional climate downscaling over Europe: Perspectives from the EURO-CORDEX community. Reg. Environ. Change 20 (2), 51–20. doi:10.1007/s10113-020-01606-9
Kendon, E. J., Ban, N., Roberts, N. M., Fowler, H. J., Roberts, M. J., Chan, S. C., et al. (2017). Do convection-permitting regional climate models improve projections of future precipitation change? Bull. Am. Meteorol. Soc. 98 (1), 79–93. doi:10.1175/BAMS-D-15-0004.1
Meinshausen, M., Nicholls, Z. R., Lewis, J., Gidden, M. J., Vogel, E., Freund, M., et al. (2020). The shared socio-economic pathway (SSP) greenhouse gas concentrations and their extensions to 2500. Geosci. Model. Dev. 13 (8), 3571–3605. doi:10.5194/gmd-13-3571-2020
Moss, R. H., Edmonds, J. A., Hibbard, K. A., Manning, M. R., Rose, S. K., van Vuuren, D. P., et al. (2010). The next generation of scenarios for climate change research and assessment. Nature 463, 747–756. doi:10.1038/nature08823
Myhre, G., Shindell, D., Bréon, F.-M., Collins, W., Fuglestvedt, J., et al. (2013). “Anthropogenic and natural radiative forcing,” in Climate change 2013: The physical science basis. Contribution of working group I to the fifth assessment report of the intergovernmental panel on climate change. Editors T. F. Stocker, D. Qin, G.-K. Plattner, M. Tignor, S. K. Allen, J. Boschunget al. (Cambridge, United Kingdom and New York, NY, USA: Cambridge University Press).
Otto-Bliesner, B. L., Brady, E. C., Fasullo, J., Jahn, A., Landrum, L., Stevenson, S., et al. (2016). Climate variability and change since 850 C.E.: An ensemble approach with the community earth system. Model. Bull. Amer. Met. Soc. 97, 735–754. doi:10.1175/BAMS-D-14-00233.1
Palmer, T. E., Booth, B. B. B., and McSweeney, C. F. (2021). How does the CMIP6 ensemble change the picture for European climate projections? Environ. Res. Lett. 16 (9), 094042. doi:10.1088/1748-9326/ac1ed9
Poschlod, B., and Ludwig, R. (2021). Internal variability and temperature scaling of future sub-daily rainfall return levels over Europe. Environ. Res. Lett. 16 (6), 064097. doi:10.1088/1748-9326/ac0849
Prein, A. F., Bukovsky, M. S., Mearns, L. O., Bruyère, C. L., and Done, J. M. (2019). Simulating North American weather types with regional climate models. Front. Environ. Sci. 7, 36. doi:10.3389/fenvs.2019.00036
Séférian, R., Nabat, P., Michou, M., Saint‐Martin, D., Voldoire, A., Colin, J., et al. (2019). Evaluation of CNRM earth‐system model, CNRM‐ESM2‐1: Role of earth system processes in present‐day and future climate. J. Adv. Model. Earth Syst. 11, 4182–4227. doi:10.1029/2019MS001791
Smith, C. J., Kramer, R. J., Myhre, G., Alterskjær, K., Collins, W., Sima, A., et al. (2020). Effective radiative forcing and adjustments in CMIP6 models. Atmos. Chem. Phys. 20, 9591–9618. doi:10.5194/acp-20-9591-2020
Sørland, S. L., Schär, C., Lüthi, D., and Kjellström, E. (2018). Bias patterns and climate change signals in GCM-RCM model chains. Environ. Res. Lett. 13 (7), 074017. doi:10.1088/1748-9326/aacc77
Takayabu, I., Kanamaru, H., Dairaku, K., Benestad, R., von Storch, H., and Christensen, J. H. (2016). Reconsidering the quality and utility of downscaling. J. Meteorological Soc. Jpn. 94, 31–45. doi:10.2151/jmsj.2015-042
Taylor, K., Stouffer, R. J., and Meehl, G. A. (2012). An overview of CMIP5 and the experiment design. Bull. Am. Meteorol. Soc. 93, 485–498. doi:10.1175/BAMS-D-11-00094.1
Tebaldi, C., Debeire, K., Eyring, V., Fischer, E., Fyfe, J., Friedlingstein, P., et al. (2021). Climate model projections from the scenario model intercomparison project (ScenarioMIP) of CMIP6. Earth Syst. Dyn. 12 (1), 253–293. doi:10.5194/esd-12-253-2021
Tebaldi, C., and Knutti, R. (2007). The use of the multi-model ensemble in probabilistic climate projections. Phil. Trans. R. Soc. A 365 (1857), 2053–2075. doi:10.1098/rsta.2007.2076
Termonia, P., Fischer, C., Bazile, E., Bouyssel, F., Brožková, R., Benard, P., et al. (2018). The ALADIN System and its canonical model configurations AROME CY41T1 and ALARO CY40T1. Geosci. Model. Dev. 11 (1), 257–281. doi:10.5194/gmd-11-257-2018
Thomas, T. S., Schlosser, C. A., Strzepek, K., Robertson, R. D., and Arndt, C. (2022). Using a large climate ensemble to assess the frequency and intensity of future extreme climate events in Southern Africa. Front. Clim. 79. doi:10.3389/fclim.2022.787721
Tokarska, K. B., Stolpe, M. B., Sippel, S., Fischer, E. M., Smith, C. J., Lehner, F., et al. (2020). Past warming trend constrains future warming in CMIP6 models. Sci. Adv. 6 (12), eaaz9549. doi:10.1126/sciadv.aaz9549
Torma, C., and Giorgi, F. (2020). On the evidence of orographical modulation of regional fine scale precipitation change signals: The Carpathians. Atmos. Sci. Lett. 21 (6), e967. doi:10.1002/asl.967
Wang, Y., Belluš, M., Ehrlich, A., Mile, M., Pristov, N., Smolikova, P., et al. (2018). 27 years of regional cooperation for limited area modelling in central Europe. Bull. Am. Meteorol. Soc. 99 (7), 1415–1432. doi:10.1175/BAMS-D-16-0321.1
Yang, H., Lu, J., Wang, Q., Shi, X., and Lohmann, G. (2022). Decoding the dynamics of poleward shifting climate zones using aqua-planet model simulations. Clim. Dyn. 58, 3513–3526. doi:10.1007/s00382-021-06112-0
Keywords: climate change projections, uncertainty, air temperature, precipitation, central Europe, annual cycle, inter-annual variability
Citation: Holtanová E, Belda M and Halenka T (2022) Projected changes in mean annual cycle of temperature and precipitation over the Czech Republic: Comparison of CMIP5 and CMIP6. Front. Earth Sci. 10:1018661. doi: 10.3389/feart.2022.1018661
Received: 13 August 2022; Accepted: 21 September 2022;
Published: 06 October 2022.
Edited by:
Jianqi Sun, Institute of Atmospheric Physics (CAS), ChinaReviewed by:
Eduardo Zorita, Helmholtz Centre for Materials and Coastal Research (HZG), GermanyHuopo Chen, Institute of Atmospheric Physics (CAS), China
Copyright © 2022 Holtanová, Belda and Halenka. This is an open-access article distributed under the terms of the Creative Commons Attribution License (CC BY). The use, distribution or reproduction in other forums is permitted, provided the original author(s) and the copyright owner(s) are credited and that the original publication in this journal is cited, in accordance with accepted academic practice. No use, distribution or reproduction is permitted which does not comply with these terms.
*Correspondence: Eva Holtanová, Eva.Holtanova@matfyz.cuni.cz